Documents
Presentation Slides
RTSNET: DEEP LEARNING AIDED KALMAN SMOOTHING
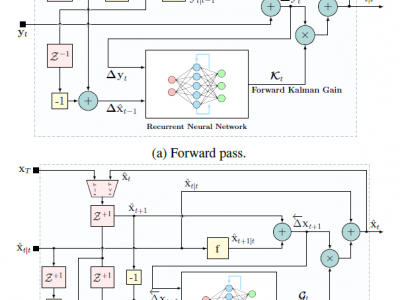
- Citation Author(s):
- Submitted by:
- Xiaoyong Ni
- Last updated:
- 5 May 2022 - 7:03am
- Document Type:
- Presentation Slides
- Document Year:
- 2022
- Event:
- Presenters:
- Xiaoyong Ni
- Paper Code:
- 2297
- Categories:
- Log in to post comments
The smoothing task is the core of many signal processing applications. It deals with the recovery of a sequence of hidden state variables from a sequence of noisy observations in a one-shot manner. In this work we propose RTSNet, a highly efficient model-based and data-driven smoothing algorithm. RTSNet integrates dedicated trainable models into the flow of the classical Rauch-Tung-Striebel (RTS) smoother, and is able to outperform it when operating under model mismatch and non-linearities while retaining its efficiency and interpretability.
Our numerical study demonstrates that although RTSNet is based on more compact neural networks, which leads to faster training and inference times, it outperforms the state-of-the-art data-driven smoother in a non-linear use case.