Documents
Poster
Solving Complex Quadratic Equations with Full-rank Random Gaussian Matrices
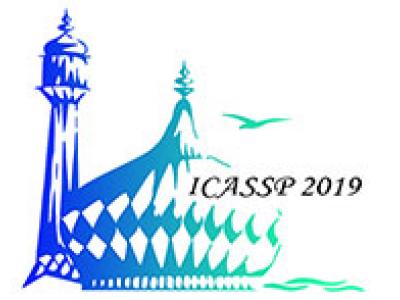
- Citation Author(s):
- Submitted by:
- Shuai Huang
- Last updated:
- 8 May 2019 - 3:11pm
- Document Type:
- Poster
- Document Year:
- 2019
- Event:
- Presenters:
- Shuai Huang
- Paper Code:
- 4850
- Categories:
- Log in to post comments
We tackle the problem of recovering a complex signal $\mathbf{x}\in\mathbb{C}^n$ from quadratic measurements of the form $y_i=\mathbf{x}^*\mathbf{A}_i\mathbf{x}$, where $\{\mathbf{A}_i\}_{i=1}^m$ is a set of complex iid standard Gaussian matrices. This non-convex problem is related to the well understood phase retrieval problem where $\mathbf{A}_i$ is a rank-1 positive semidefinite matrix. Here we study a general full-rank case which models a number of key applications such as molecular geometry recovery from distance distributions and compound measurements in phaseless diffractive imaging. Most prior work either addresses the rank-1 case or focuses on real measurements. The several papers that address the full-rank complex case adopt the semidefinite relaxation approach and are thus computationally demanding. In this paper we propose a method based on the standard framework comprising a spectral initialization followed by iterative gradient descent updates. We prove that when the number of measurements exceeds the signal's length by some constant factor, a globally optimal solution can be recovered from complex quadratic measurements with high probability. Numerical experiments on simulated data corroborate our theoretical analysis.