Documents
Presentation Slides
Streaming Multi-Speaker ASR with RNN-T
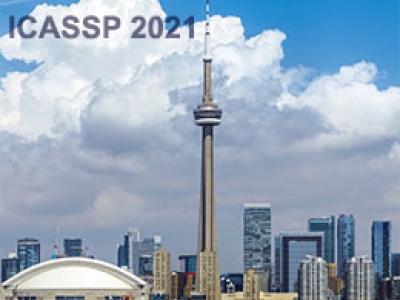
- Citation Author(s):
- Submitted by:
- Yulan Liu
- Last updated:
- 22 June 2021 - 11:11am
- Document Type:
- Presentation Slides
- Document Year:
- 2021
- Event:
- Paper Code:
- 2057
- Categories:
- Log in to post comments
Recent research shows end-to-end ASR systems can recognize overlapped speech from multiple speakers. However, all published works have assumed no latency constraints during inference, which does not hold for most voice assistant inter- actions. This work focuses on multi-speaker speech recognition based on a recurrent neural network transducer (RNN-T) that has been shown to provide high recognition accuracy at a low latency online recognition regime. We investigate two approaches to multi-speaker model training of the RNN-T: deterministic output-target assignment and permutation invariant training. We show that guiding separation with speaker order labels in the former case enhances the high-level speaker tracking capability of RNN-T. Apart from that, with multi- style training on single- and multi-speaker utterances, the resulting models gain robustness against ambiguous numbers of speakers during inference. Our best model achieves a WER of 10.2% on simulated 2-speaker LibriSpeech data, which is competitive with the previously reported state-of-the-art non- streaming model (10.3%), while the proposed model could be directly applied for streaming applications.