Documents
Presentation Slides
A study of the robustness of raw waveform based speaker embeddings under mismatched conditions
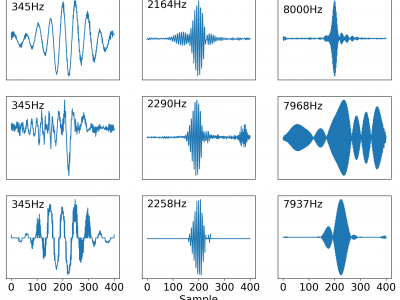
- Citation Author(s):
- Submitted by:
- Ge Zhu
- Last updated:
- 5 May 2022 - 1:16pm
- Document Type:
- Presentation Slides
- Document Year:
- 2022
- Event:
- Presenters:
- Ge Zhu
- Paper Code:
- 5429
- Categories:
- Log in to post comments
In this paper, we conduct a cross-dataset study on parametric and non-parametric raw-waveform based speaker embeddings through speaker verification experiments. In general, we observe a more significant performance degradation of these raw-waveform systems compared to spectral based systems. We then propose two strategies to improve the performance of raw-waveform based systems on cross-dataset tests. The first strategy is to change the real-valued filters into analytic filters to ensure shift-invariance. The second strategy is to apply variational dropout to non-parametric filters to prevent them from overfitting irrelevant nuance features. By combining these strategies, we achieve results comparable to spectral based systems on both the VoxCeleb and VOiCEs datasets. Futhermore, we demonstrate that the learned filters carry little noise compared to existing non-parametric learnable front-ends.