Documents
Whitepaper
Transfer Learning for Robust Low-Resource Children's Speech ASR with Transformers and Source-Filter Warping
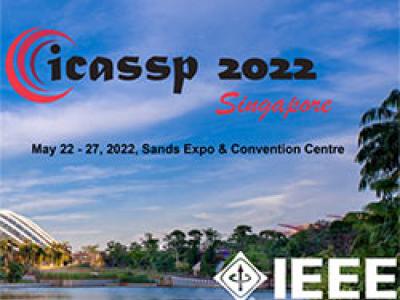
- Citation Author(s):
- Submitted by:
- Jenthe Thienpondt
- Last updated:
- 5 May 2022 - 5:10am
- Document Type:
- Whitepaper
- Document Year:
- 2022
- Event:
- Presenters:
- Jenthe Thienpondt
- Categories:
- Log in to post comments
Automatic Speech Recognition (ASR) systems are known to exhibit difficulties when transcribing children's speech. This can mainly be attributed to the absence of large children’s speech corpora to train robust ASR models and the resulting domain mismatch when decoding children’s speech with systems trained on adult data. In this paper, we propose multiple enhancements to alleviate these issues. First, we propose a data augmentation technique based on the source-filter model of speech to close the domain gap between adult and children's speech. This enables us to leverage the data availability of adult speech corpora by making these samples perceptually similar to children's speech. Second, using this augmentation strategy, we apply transfer learning on a Transformer model pre-trained on adult data. This model follows the recently introduced XLS-R architecture, a wav2vec 2.0 model pre-trained on several cross-lingual adult speech corpora to learn general and robust acoustic frame-level representations. Adopting this model for the ASR task using adult data augmented with the proposed source-filter warping strategy and a limited amount of in-domain children’s speech significantly outperforms previous state-of-the-art results on the PF-STAR British English Children’s Speech corpus with a 4.86% WER on the official test set.