Documents
Poster
Two-Stage Noise Aware Training Using Asymmetric Deep Denoising Autoencoder
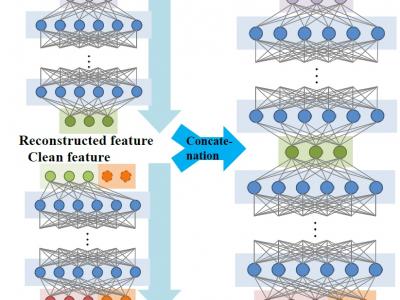
- Citation Author(s):
- Submitted by:
- KANGHYUN LEE
- Last updated:
- 17 March 2016 - 1:45am
- Document Type:
- Poster
- Document Year:
- 2016
- Event:
- Presenters:
- Kang Hyun Lee
- Categories:
- Log in to post comments
Ever since the deep neural network (DNN)-based acoustic model appeared, the recognition performance of automatic peech recognition has been greatly improved. Due to this achievement, various researches on DNN-based technique for noise robustness are also in progress. Among these approaches, the noise-aware training (NAT) technique which aims to improve the inherent robustness of DNN using noise estimates has shown remarkable performance. However, despite the great performance, we cannot be certain whether NAT is an optimal method for sufficiently utilizing the inherent robustness of DNN. In this paper, we propose a novel technique which helps the DNN to address the complex connection between the input and target vectors of NAT smoothly. The proposed method outperformed the conventional NAT in Aurora-5 task.