Documents
Poster
Unimodal Aggregation for CTC-based Speech Recognition
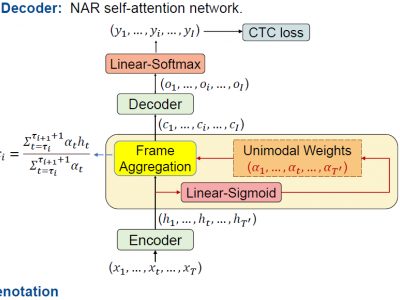
- DOI:
- 10.60864/h3jx-0106
- Citation Author(s):
- Submitted by:
- Ying Fang
- Last updated:
- 2 April 2024 - 3:01am
- Document Type:
- Poster
- Document Year:
- 2024
- Event:
- Presenters:
- Ying Fang
- Paper Code:
- SLP-P4.7
- Categories:
- Keywords:
- Log in to post comments
This paper works on non-autoregressive automatic speech recognition. A unimodal aggregation (UMA) is proposed to segment and integrate the feature frames that belong to the same text token, and thus to learn better feature representations for text tokens. The frame-wise features and weights are both derived from an encoder. Then, the feature frames with unimodal weights are integrated and further processed by a decoder. Connectionist temporal classification (CTC) loss is applied for training. Compared to the regular CTC, the proposed method learns better feature representations and shortens the sequence length, resulting in lower recognition error and computational complexity. Experiments on three Mandarin datasets show that UMA demonstrates superior or comparable performance to other advanced non-autoregressive methods, such as self-conditioned CTC. Moreover, by integrating self-conditioned CTC into the proposed framework, the performance can be further noticeably improved.