Documents
Poster
WAV2VEC-SWITCH: CONTRASTIVE LEARNING FROM ORIGINAL-NOISY SPEECH PAIRS FOR ROBUST SPEECH RECOGNITION
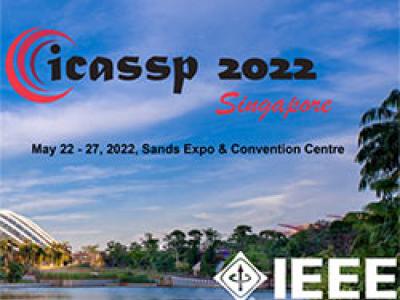
- Citation Author(s):
- Submitted by:
- Yiming Wang
- Last updated:
- 4 May 2022 - 8:20pm
- Document Type:
- Poster
- Document Year:
- 2022
- Event:
- Presenters:
- Yiming Wang
- Paper Code:
- SPE-38.3
- Categories:
- Keywords:
- Log in to post comments
The goal of self-supervised learning (SSL) for automatic speech recognition (ASR) is to learn good speech representations from a large amount of unlabeled speech for the downstream ASR task. However, most SSL frameworks do not consider noise robustness which is crucial for real-world applications. In this paper we propose wav2vec-Switch, a method to encode noise robustness into contextualized representations of speech via contrastive learning. Specifically, we feed original-noisy speech pairs simultaneously into the wav2vec 2.0 network. In addition to the existing contrastive learning task, we switch the quantized representations of the original and noisy speech as additional prediction targets of each other. By doing this, it enforces the network to have consistent predictions for the original and noisy speech, thus allows to learn contextualized representation with noise robustness. Our experiments on synthesized and real noisy data show the effectiveness of our method: it achieves 2.9--4.9% relative word error rate (WER) reduction on the synthesized noisy LibriSpeech data without deterioration on the original data, and 5.7% on CHiME-4 real 1-channel noisy data compared to a data augmentation baseline even with a strong language model for decoding. Our results on CHiME-4 can match or even surpass those with well-designed speech enhancement components.