Documents
Presentation Slides
Verifying the Long-range Dependency of RNN Language Models
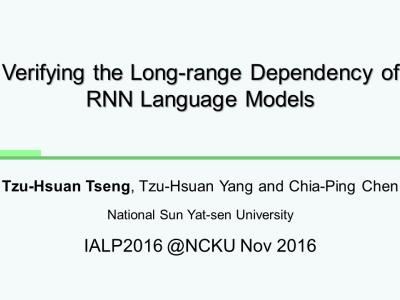
- Citation Author(s):
- Submitted by:
- Tzu-Hsuan Tseng
- Last updated:
- 21 November 2016 - 10:24am
- Document Type:
- Presentation Slides
- Document Year:
- 2016
- Event:
- Presenters:
- Tzu-Hsuan, Tseng
- Paper Code:
- 41
- Categories:
- Keywords:
- Log in to post comments
It has been argued that recurrent neural network language models are better in capturing long-range dependency than n-gram language models. In this paper, we attempt to verify this claim by investigating the prediction accuracy and the perplexity of these language models as a function of word position, i.e., the position of a word in a sentence. It is expected that as word position increases, the advantage of using recurrent neural network language models over n-gram language models will become more and more evident. On the text corpus of Penn Tree Bank (PTB), a recurrent neural network language model outperforms a trigram language model in both perplexity and word prediction. However, on the AMI meeting corpus, a trigram outperforms a recurrent neural network language model.