This paper describes a model to address the task of named-entity recognition on Indonesian microblog messages due to its usefulness for higher-level tasks or text mining applications on Indonesian microblogs. We view our task as a sequence labeling problem using machine learning approach. We also propose various word-level and orthographic features, including the ones that are specific to the Indonesian language. Finally, in our experiment, we compared our model with a baseline model previously proposed for Indonesian formal documents, instead of microblog messages.
- Categories:
- Read more about An Initial Study of Indonesian Semantic Role Labelingand Its Application on Event Extraction
- Log in to post comments
Semantic role labeling (SRL) is a task to as- sign semantic role labels to sentence elements. This pa- per describes the initial development of an Indonesian semantic role labeling system and its application to extract event information from Tweets. We compare two feature types when designing the SRL systems: Word-to-Word and Phrase-to-Phrase. Our experiments showed that the Word- to-Word feature approach outperforms the Phrase-to-Phrase approach. The application of the SRL system to an event extraction problem resulted overlap-based accuracy of 0.94 for the actor identification.
- Categories:
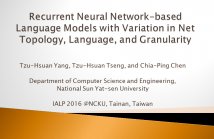
- Read more about Recurrent Neural Network-based Language Models with Variation in Net Topology, Language, and Granularity
- Log in to post comments
In this paper, we study language models based on recurrent neural networks on three databases in two languages. We implement basic recurrent neural networks (RNN) and refined RNNs with long short-term memory (LSTM) cells. We use the corpora of Penn Tree Bank (PTB) and AMI in English, and the Academia Sinica Balanced Corpus (ASBC) in Chinese. On ASBC, we investigate wordbased and character-based language models. For characterbased language models, we look into the cases where the inter-word space is treated or not treated as a token.
40_RNN.pdf

- Categories:
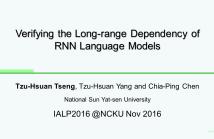
It has been argued that recurrent neural network language models are better in capturing long-range dependency than n-gram language models. In this paper, we attempt to verify this claim by investigating the prediction accuracy and the perplexity of these language models as a function of word position, i.e., the position of a word in a sentence. It is expected that as word position increases, the advantage of using recurrent neural network language models over n-gram language models will become more and more evident.
- Categories: