Documents
Presentation Slides
SANDGLASSET: A LIGHT MULTI-GRANULARITY SELF-ATTENTIVE NETWORK FOR TIME-DOMAIN SPEECH SEPARATION
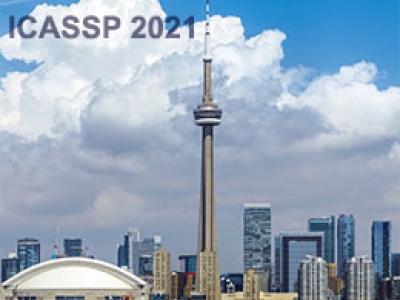
- Citation Author(s):
- Submitted by:
- Max W. Y. Lam
- Last updated:
- 22 June 2021 - 3:40am
- Document Type:
- Presentation Slides
- Document Year:
- 2021
- Event:
- Presenters:
- Max W. Y. Lam
- Categories:
- Log in to post comments
One of the leading single-channel speech separation (SS) models is based on a TasNet with a dual-path segmentation technique, where the size of each segment remains unchanged throughout all layers. In contrast, our key finding is that multi-granularity features are essential for enhancing contextual modeling and computational efficiency. We introduce a self-attentive network with a novel sandglass-shape, namely Sandglasset, which advances the state-of-the-art (SOTA) SS performance at significantly smaller model size and computational cost. Forward along each block inside Sandglasset, the temporal granularity of the features gradually becomes coarser until reaching half of the network blocks, and then successively turns finer towards the raw signal level. We also unfold that residual connections between features with the same granularity are critical for preserving information after passing through the bottleneck layer. Experiments show our Sandglasset with only 2.3M parameters has achieved the best results on two benchmark SS datasets -- WSJ0-2mix and WSJ0-3mix, where the SI-SNRi scores have been improved by absolute 0.8 dB and 2.4 dB, respectively, comparing to the prior SOTA results.