Documents
Poster
Deep Convolutional and Recurrent Networks for Polyphonic Instrument Classification from Monophonic Raw Audio Waveforms
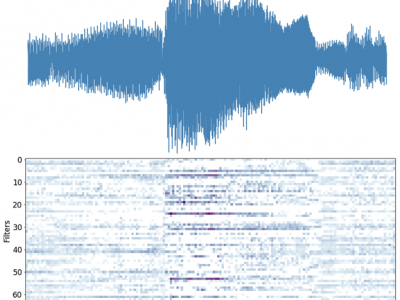
- Citation Author(s):
- Submitted by:
- Kleanthis Avramids
- Last updated:
- 22 June 2021 - 8:29am
- Document Type:
- Poster
- Document Year:
- 2021
- Event:
- Presenters:
- Kleanthis Avramidis
- Paper Code:
- MLSP-10.4
- Categories:
- Log in to post comments
Sound Event Detection and Audio Classification tasks are traditionally addressed through time-frequency representations of audio signals such as spectrograms. However, the emergence of deep neural networks as efficient feature extractors has enabled the direct use of audio signals for classification purposes. In this paper, we attempt to recognize musical instruments in polyphonic audio by only feeding their raw waveforms into deep learning models. Various recurrent and convolutional architectures incorporating residual connections are examined and parameterized in order to build end-to-end classifiers with low computational cost and only minimal preprocessing. We obtain competitive classification scores and useful instrument-wise insight through the IRMAS test set, utilizing a parallel CNN-BiGRU model with multiple residual connections, while maintaining a significantly reduced number of trainable parameters.