Documents
Poster
Restoration of Time-Varying Graph Signals using Deep Algorithm Unrolling
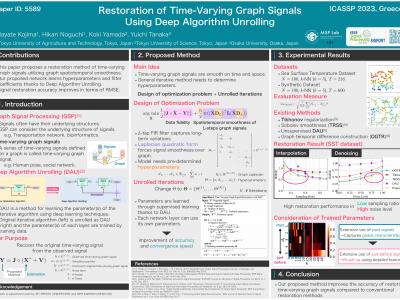
- DOI:
- 10.60864/qjrc-qw76
- Citation Author(s):
- Submitted by:
- Hayate KOJIMA
- Last updated:
- 17 November 2023 - 12:08pm
- Document Type:
- Poster
- Document Year:
- 2023
- Event:
- Presenters:
- Hayate Kojima
- Paper Code:
- SPTM-P5.11
- Categories:
- Log in to post comments
In this paper, we propose a restoration method of time-varying graph signals, i.e., signals on a graph whose signal values change over time, using deep algorithm unrolling. Deep algorithm unrolling is a method that learns parameters in an iterative optimization algorithm with deep learning techniques. It is expected to improve convergence speed and accuracy while the iterative steps are still interpretable. In the proposed method, the minimization problem is formulated so that the time-varying graph signal is smooth both in time and spatial domains. The internal parameters, i.e., time domain FIR filters and regularization parameters, are learned from training data. Experimental results using synthetic data and real sea surface temperature data show that the proposed method improves signal reconstruction accuracy compared to several existing time-varying graph signal reconstruction methods.