Documents
Research Manuscript
NOMAD: Non-Matching Audio Distance
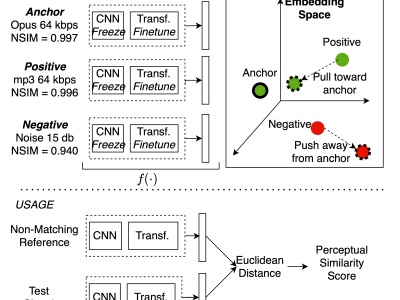
- Citation Author(s):
- Submitted by:
- Alessandro Ragano
- Last updated:
- 13 April 2024 - 4:47am
- Document Type:
- Research Manuscript
- Document Year:
- 2024
- Event:
- Presenters:
- Alessandro Ragano
- Paper Code:
- AASP-P6.5
- Categories:
- Log in to post comments
This paper presents NOMAD (Non-Matching Audio Distance), a differentiable perceptual similarity metric that measures the distance of a degraded signal against non-matching references. The proposed method is based on learning deep feature embeddings via a triplet loss guided by the Neurogram Similarity Index Measure (NSIM) to capture degradation intensity. During inference, the similarity score between any two audio samples is computed through Euclidean distance of their embeddings. NOMAD is fully unsupervised and can be used in general perceptual audio tasks for audio analysis e.g. quality assessment and generative tasks such as speech enhancement and speech synthesis. The proposed method is evaluated with 3 tasks. Ranking degradation intensity, predicting speech quality, and as a loss function for speech enhancement. Results indicate NOMAD outperforms other non-matching reference approaches in both ranking degradation intensity and quality assessment, exhibiting competitive performance with full-reference audio metrics. NOMAD demonstrates a promising technique that mimics human capabilities in assessing audio quality with non-matching references to learn perceptual embeddings without the need for human-generated labels.