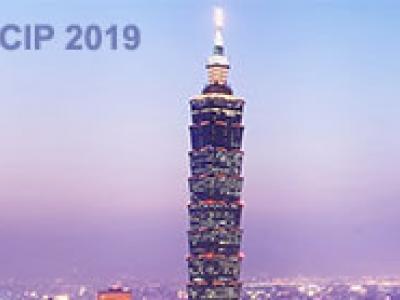
- Read more about Evolutionary Camera Pose Estimation of a Multi-Camera Setup for Computed Tomography
- Log in to post comments
- Categories:
- Read more about AUTODEPTH: SINGLE IMAGE DEPTH MAP ESTIMATION VIA RESIDUAL CNN ENCODER-DECODER AND STACKED HOURGLASS
- Log in to post comments
We address the task of estimating depth from a single intensity image via a novel convolutional neural network (CNN) encoder-decoder architecture, which learns the depth information using example pairs of color images and their corresponding depth maps. The proposed model integrates residual connections within pooling and up-sampling layers, and hourglass networks which operate on the encoded features, thus processing these at various scales. Furthermore, the model is optimized under the constraints of perceptual as well as the mean squared error loss.
eposter_autodepth.pdf

- Categories:
- Read more about ACCURATE MESH-BASED ALIGNMENT FOR GROUND AND AERIAL MULTI-VIEW STEREO MODELS
- Log in to post comments
We propose a method for accurate alignment of ground and aerial multi-view stereo (MVS) models. We achieve this goal by reconstructing the surface meshes from MVS point clouds generated by aerial and ground images respectively, and then iteratively removing the gap between them. The key issue is how to establish reliable correspondences between two meshes.
- Categories: