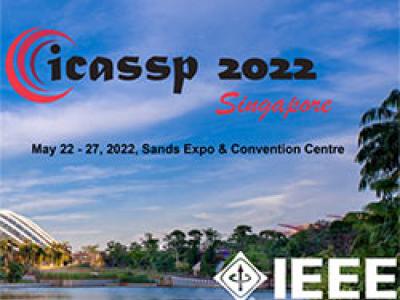
- Read more about A TEST FOR CONDITIONAL CORRELATION BETWEEN RANDOM VECTORS BASED ON WEIGHTED U-STATISTICS
- Log in to post comments
This article explores U-Statistics as a tool for testing conditional correlation between two multivariate sources with respect to a potential confounder. The proposed approach is effectively an instance of weighted U-Statistics and does not impose any statistical model on the processed data, in contrast to other well-known techniques that assume Gaussianity. By avoiding determinants and inverses, the method presented displays promising robustness in small-sample regimes. Its performance is evaluated numerically through its MSE and ROC curves.
- Categories:
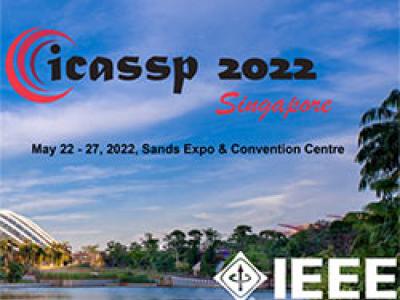
- Read more about A TEST FOR CONDITIONAL CORRELATION BETWEEN RANDOM VECTORS BASED ON WEIGHTED U-STATISTICS
- Log in to post comments
This article explores U-Statistics as a tool for testing conditional correlation between two multivariate sources with respect to a potential confounder. The proposed approach is effectively an instance of weighted U-Statistics and does not impose any statistical model on the processed data, in contrast to other well-known techniques that assume Gaussianity. By avoiding determinants and inverses, the method presented displays promising robustness in small-sample regimes. Its performance is evaluated numerically through its MSE and ROC curves.
- Categories:
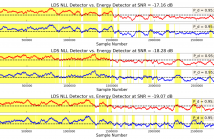
A machine learning approach to detecting unknown signals in time-correlated noise is presented. In the proposed approach, a linear dynamical system (LDS) model is trained to represent the background noise via expectation-maximization (EM). The negative log-likelihood (NLL) of test data under the learned background noise LDS is computed via the Kalman filter recursions, and an unknown signal is detected if the NLL exceeds a threshold. The proposed detection scheme is derived as a generalized likelihood ratio test (GLRT) for an unknown deterministic signal in LDS noise.
poster_mlsp.pdf

- Categories: