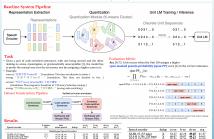
- Read more about Zero Resource Code-switched Speech Benchmark Using Speech Utterance Pairs For Multiple Spoken Languages
- Log in to post comments
We introduce a new zero resource code-switched speech benchmark designed to directly assess the code-switching capabilities of self-supervised speech encoders. We showcase a baseline system of language modeling on discrete units to demonstrate how the code-switching abilities of speech encoders can be assessed in a zero-resource manner. Our experiments encompass a variety of well-known speech encoders, including Wav2vec 2.0, HuBERT, XLSR, etc. We examine the impact of pre-training languages and model size on benchmark performance.
- Categories:
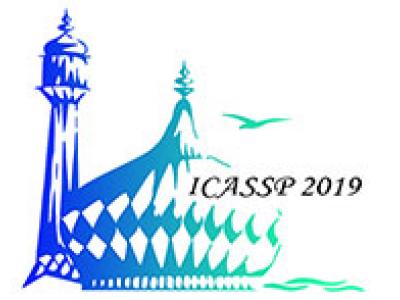
- Read more about ONLINE SINGING VOICE SEPARATION USING A RECURRENT ONE-DIMENSIONAL U-NET TRAINED WITH DEEP FEATURE LOSSES
- Log in to post comments
This paper proposes an online approach to the singing voice separation problem. Based on a combination of one-dimensional convolutional layers along the frequency axis and recurrent layers to enforce temporal coherency, state-of-the-art performance is achieved. The concept of using deep features in the loss function to guide training and improve the model’s performance is also investigated.
- Categories: