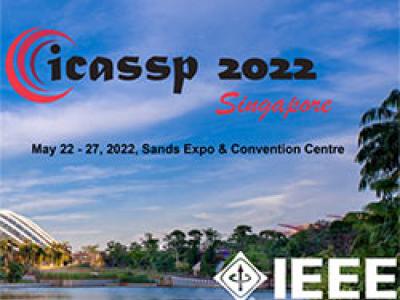
- Read more about "DYNAMIC RESOURCEOPTIMIZATION FORADAPTIVE FEDERATED LEARNING EMPOWERED BY RECONFIGURABLE INTELLIGENT SURFACES" Poster
- Log in to post comments
The aim of this work is to propose a novel dynamic resource allocation strategy for adaptive Federated Learning (FL), in the context of beyond 5G networks endowed with Reconfigurable Intelligent Surfaces (RISs). Due to time-varying wireless channel conditions, communication resources (e.g., set of transmitting devices, transmit powers, bits), computation parameters (e.g., CPU cycles at devices and at server) and RISs reflectivity must be optimized in each communication round, in order to strike the best trade-off between power, latency, and performance of the FL task.
- Categories:
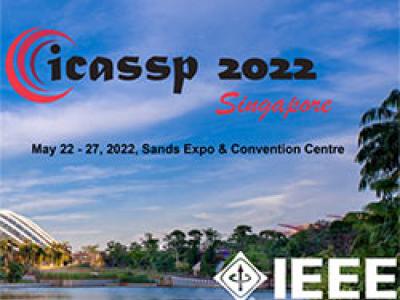
- Read more about "DYNAMIC RESOURCEOPTIMIZATION FORADAPTIVE FEDERATED LEARNING EMPOWERED BY RECONFIGURABLE INTELLIGENT SURFACES" Slides
- Log in to post comments
The aim of this work is to propose a novel dynamic resource allocation strategy for adaptive Federated Learning (FL), in the context of beyond 5G networks endowed with Reconfigurable Intelligent Surfaces (RISs). Due to time-varying wireless channel conditions, communication resources (e.g., set of transmitting devices, transmit powers, bits), computation parameters (e.g., CPU cycles at devices and at server) and RISs reflectivity must be optimized in each communication round, in order to strike the best trade-off between power, latency, and performance of the FL task.
- Categories:
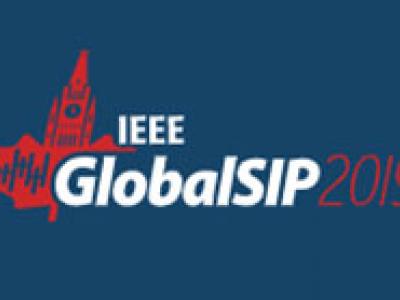
- Read more about MAC ID Spoofing-Resistant Radio Fingerprinting
- Log in to post comments
We explore the resistance of deep learning methods for radio fingerprinting to MAC ID spoofing. We demonstrate that classifying transmission slices enables classification of a transmission with a fixed-length input deep classifier, enhances shift-invariance, and, most importantly, makes the classifier resistant to MAC ID spoofing. This is a consequence of the fact that the classifier does not learn to use the MAC ID to classifying among transmissions, but relies on other inherent discriminating signals, e.g., device imperfections.
- Categories: