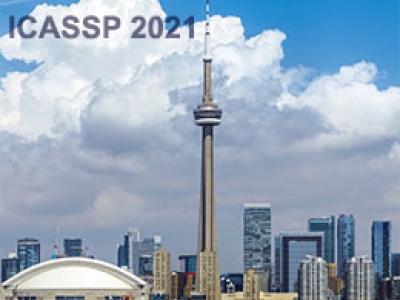
- Read more about Audio-Visual Speech Inpainting with Deep Learning
- Log in to post comments
In this paper, we present a deep-learning-based framework for audio-visual speech inpainting, i.e. the task of restoring the missing parts of an acoustic speech signal from reliable audio context and uncorrupted visual information. Recent work focuses solely on audio-only methods and they generally aim at inpainting music signals, which show highly different structure than speech. Instead, we inpaint speech signals with gaps ranging from 100 ms to 1600 ms to investigate the contribution that vision can provide for gaps of different duration.
- Categories:
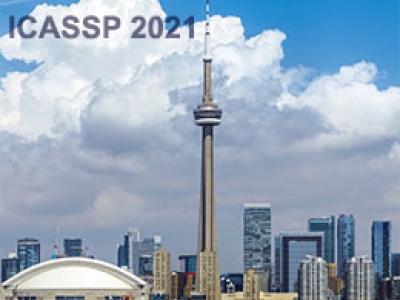
- Read more about SANDGLASSET: A LIGHT MULTI-GRANULARITY SELF-ATTENTIVE NETWORK FOR TIME-DOMAIN SPEECH SEPARATION
- Log in to post comments
One of the leading single-channel speech separation (SS) models is based on a TasNet with a dual-path segmentation technique, where the size of each segment remains unchanged throughout all layers. In contrast, our key finding is that multi-granularity features are essential for enhancing contextual modeling and computational efficiency. We introduce a self-attentive network with a novel sandglass-shape, namely Sandglasset, which advances the state-of-the-art (SOTA) SS performance at significantly smaller model size and computational cost.
- Categories:
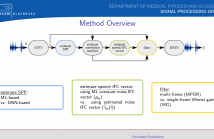
- Read more about DNN-Based Speech Presence Probability Estimation for Multi-Frame Single-Microphone Speech Enhancement
- Log in to post comments
Multi-frame approaches for single-microphone speech enhancement, e.g., the multi-frame minimum-power-distortionless-response (MFMPDR) filter, are able to exploit speech correlations across neighboring time frames. In contrast to single-frame approaches such as the Wiener gain, it has been shown that multi-frame approaches achieve a substantial noise reduction with hardly any speech distortion, provided that an accurate estimate of the correlation matrices and especially the speech interframe correlation (IFC) vector is available.
- Categories:
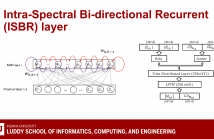
- Read more about Monaural Speech Enhancement Using Intra-Spectral Recurrent Layers In The Magnitude And Phase Responses
- Log in to post comments
Speech enhancement has greatly benefited from deep learning. Currently, the best performing deep architectures use long short-term memory (LSTM) recurrent neural networks (RNNs) to model short and long temporal dependencies. These approaches, however, underutilize or ignore spectral-level dependencies within the magnitude and phase responses, respectively. In this paper, we propose a deep learning architecture that leverages both temporal and spectral dependencies within the magnitude and phase responses.
- Categories:
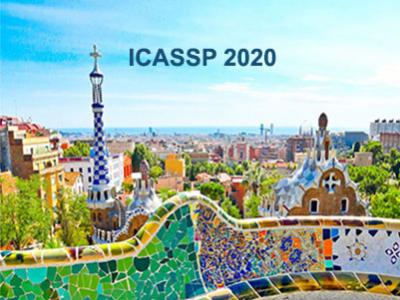
- Read more about A RETURN TO DEREVERBERATION IN THE FREQUENCY DOMAIN USING A JOINT LEARNING APPROACH
- Log in to post comments
Dereverberation is often performed in the time-frequency domain using mostly deep learning approaches. Time-frequency domain processing, however, may not be necessary when reverberation is modeled by the convolution operation. In this paper, we investigate whether deverberation can be effectively performed in the frequency-domain by estimating the complex frequency response of a room impulse response. More specifically, we develop a joint learning framework that uses frequency-domain estimates of the late reverberant response to assist with estimating the direct and early response.
- Categories:
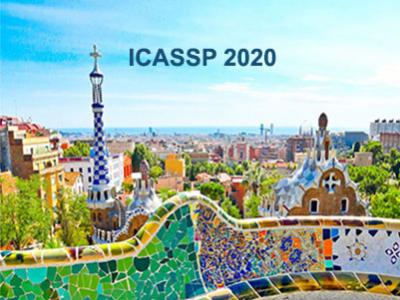
- Read more about Self-Supervised Denoising Autoencoder with Linear Regression Decoder for Speech Enhancement
- Log in to post comments
Nonlinear spectral mapping-based models based on supervised learning have successfully applied for speech enhancement. However, as supervised learning approaches, a large amount of labelled data (noisy-clean speech pairs) should be provided to train those models. In addition, their performances for unseen noisy conditions are not guaranteed, which is a common weak point of supervised learning approaches. In this study, we proposed an unsupervised learning approach for speech enhancement, i.e., denoising autoencoder with linear regression decoder (DAELD) model for speech enhancement.
PPT_DAELD.pdf

- Categories:
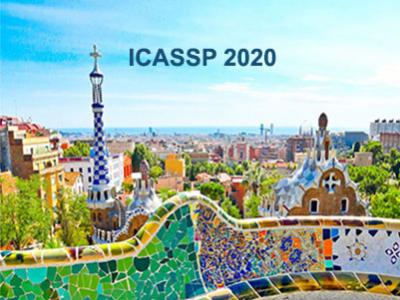
- Read more about CLCNet: Deep learning-based noise reduction for hearing aids using Complex Linear Coding
- Log in to post comments
Noise reduction is an important part of modern hearing aids and is included in most commercially available devices. Deep learning-based state-of-the-art algorithms, however, either do not consider real-time and frequency resolution constrains or result in poor quality under very noisy conditions.To improve monaural speech enhancement in noisy environments, we propose CLCNet, a framework based on complex valued linear coding. First, we define complex linear coding (CLC) motivated by linear predictive coding (LPC) that is applied in the complex frequency domain.
- Categories:
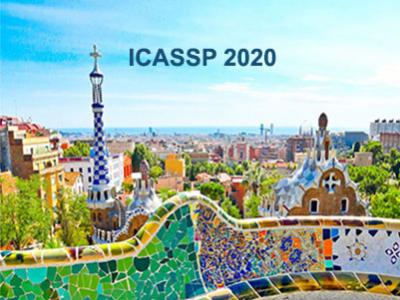
- Read more about ENHANCING END-TO-END MULTI-CHANNEL SPEECH SEPARATION VIA SPATIAL FEATURE LEARNING
- Log in to post comments
Hand-crafted spatial features (e.g., inter-channel phase difference, IPD) play a fundamental role in recent deep learning based multi-channel speech separation (MCSS) methods. However, these manually designed spatial features are hard to incorporate into the end-to-end optimized MCSS framework. In this work, we propose an integrated architecture for learning spatial features directly from the multi-channel speech waveforms within an end-to-end speech separation framework. In this architecture, time-domain filters spanning signal channels are trained to perform adaptive spatial filtering.
- Categories:
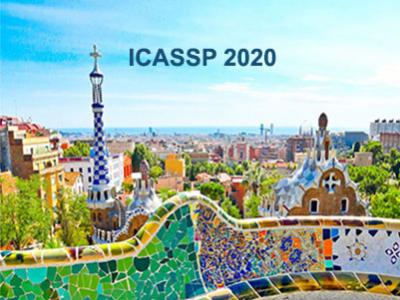
- Categories: