Documents
Presentation Slides
4D Convolutional Neural Networks for Multi Spectral and Multi Temporal Remote Sensing Data Classification
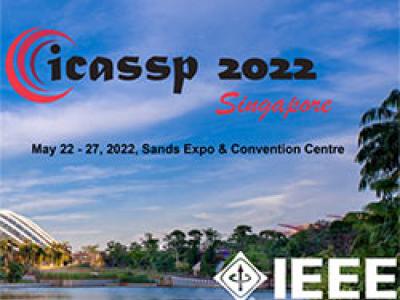
- Citation Author(s):
- Submitted by:
- Michalis Gianno...
- Last updated:
- 14 May 2022 - 8:48am
- Document Type:
- Presentation Slides
- Document Year:
- 2022
- Event:
- Presenters:
- Michalis Giannopoulos
- Paper Code:
- CI-2.4
- Categories:
- Keywords:
- Log in to post comments
Multi-temporal remotely sensed observations acquired by multi-spectral sensors contain a wealth of information related to the Earth’s state. Deep learning methods have demonstrated a great potential in analyzing such observations. Traditional 2D and 3D approaches are unable to effectively extract valuable information encoded across all available dimensions. In this work, we propose the extension of current fully-convolutional models for multi-temporal remote sensing data classification to their high-dimensional analogs, which can naturally capture multi-dimensional dependencies and correlations. Experimental analysis on recently released observations from Landsat-8 reveals that our proposed high-dimensional fully-convolutional approach exhibits a clear classification performance improvement over state of the art low-order fully-convolutional models.