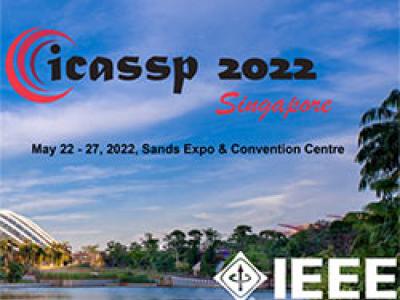
- Read more about 4D Convolutional Neural Networks for Multi Spectral and Multi Temporal Remote Sensing Data Classification
- Log in to post comments
Multi-temporal remotely sensed observations acquired by multi-spectral sensors contain a wealth of information related to the Earth’s state. Deep learning methods have demonstrated a great potential in analyzing such observations. Traditional 2D and 3D approaches are unable to effectively extract valuable information encoded across all available dimensions.
- Categories:
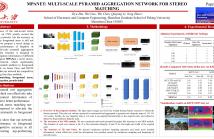
At present, the performance of the end-to-end stereo matching networks based on CNN greatly exceed the traditional stereo matching networks, but the accuracy in those ill-posed regions like foreground areas is still not optimistic. In this paper, we propose a novel design to improve the prediction performance of disparity in foreground. First, a multi-scale pyramid aggregation module with hourglass-like structure is designed to effectively utilize the aggregation information of different scales.
- Categories:
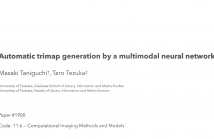
In many of the existing alpha matting implementations, an intermediate representation called a trimap needs to be created manually. To automate the process, we propose a generic neural network for trimap generation based on saliency map detection. Our model multi-modally learns a saliency map and a trimap simultaneously. Because of this structure, the network focuses on reducing the error of the trimap especially within the areas with high salience.
- Categories: