Documents
Presentation Slides
FAST: Flow-Assisted Shearlet Transform for Densely-sampled Light Field Reconstruction
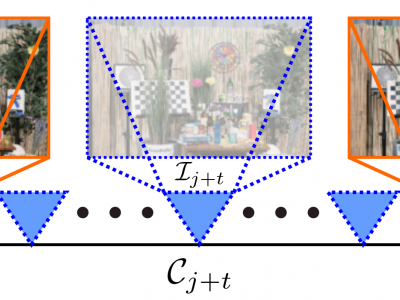
- Citation Author(s):
- Submitted by:
- Yuan Gao
- Last updated:
- 16 September 2019 - 12:24pm
- Document Type:
- Presentation Slides
- Document Year:
- 2019
- Event:
- Paper Code:
- WP.L5.2
- Categories:
- Log in to post comments
Shearlet Transform (ST) is one of the most effective methods for Densely-Sampled Light Field (DSLF) reconstruction from a Sparsely-Sampled Light Field (SSLF). However, ST requires a precise disparity estimation of the SSLF. To this end, in this paper a state-of-the-art optical flow method, i.e. PWC-Net, is employed to estimate bidirectional disparity maps between neighboring views in the SSLF. Moreover, to take full advantage of optical flow and ST for DSLF reconstruction, a novel learning-based method, referred to as Flow-Assisted Shearlet Transform (FAST), is proposed in this paper. Specifically, FAST consists of two deep convolutional neural networks, i.e. disparity refinement network and view synthesis network, which fully leverage the disparity information to synthesize novel views via warping and blending and to improve the novel view synthesis performance of ST. Experimental results demonstrate the superiority of the proposed FAST method over the other state-of-the-art DSLF reconstruction methods on nine challenging real-world SSLF sub-datasets with large disparity ranges (up to 26 pixels).