Documents
Poster
MaskDUL: Data Uncertainty Learning in Masked Face Recognition
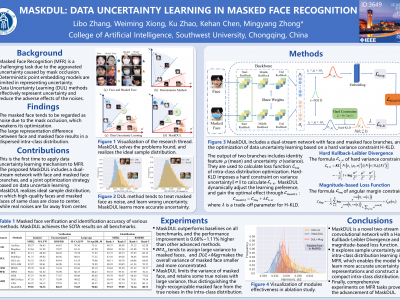
- Citation Author(s):
- Submitted by:
- libo zhang
- Last updated:
- 27 May 2023 - 11:09am
- Document Type:
- Poster
- Document Year:
- 2023
- Event:
- Presenters:
- Libo Zhang
- Paper Code:
- IVMSP-P8.4
- Categories:
- Keywords:
- Log in to post comments
Since mask occlusion causes plentiful loss of facial feature, Masked Face Recognition (MFR) is a challenging image processing task, and the recognition results are susceptible to noise. However, existing MFR methods are mostly deterministic point embedding models, which are limited in representing noise images. Moreover, Data Uncertainty Learning (DUL) fails to achieve reasonable performance in MFR. Therefore, we propose a novel two-stream convolutional network, masked face data uncertainty learning (MaskDUL), that solves the problems by sampling uncertainty and intra-class distribution learning in MFR. Specifically, a Hard Kullback-Leibler Divergence (H-KLD) method is proposed to serve as an adaptive variance regularizer and a magnitude-based module is adopted to adaptively adjust the angular margin of different samples. Finally, insightful evaluation demonstrates the effectiveness and robustness of our MaskDUL.
Poster.pdf
