Documents
Presentation Slides
Minimax Active Learning via Minimal Model Capacity
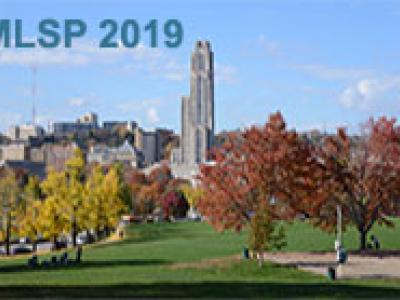
- Citation Author(s):
- Submitted by:
- Shachar Shayovitz
- Last updated:
- 16 October 2019 - 4:02pm
- Document Type:
- Presentation Slides
- Document Year:
- 2019
- Event:
- Presenters:
- Shachar Shayovitz
- Categories:
- Log in to post comments
Active learning is a form of machine learning which combines supervised learning and feedback to minimize the training set size, subject to low generalization errors. Since direct optimization of the generalization error is difficult, many heuristics have been developed which lack a firm theoretical foundation. In this paper, a new information theoretic criterion is proposed based on a minimax log-loss regret formulation of the active learning problem. In the first part of this paper, a Redundancy Capacity theorem for active learning is derived along with an optimal learner. Building on this, a new active learning criterion is proposed which naturally induces an exploration - exploitation trade-off in feature selection. In the second part, the linear separator hypotheses class with additive label noise is considered and a low complexity algorithm is proposed which optimizes the active learning criterion from the first part. This greedy algorithm is based on the Posterior Matching scheme for communication with feedback and is shown that for BSC and BEC label noise, the proposed information theoretic criterion decays at an exponential rate.