Documents
Poster
ModEFormer: Modality-preserving embedding for audio-video synchronization using transformers
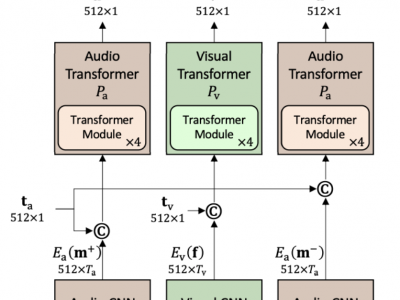
- Citation Author(s):
- Submitted by:
- Akash Gupta
- Last updated:
- 26 May 2023 - 6:30pm
- Document Type:
- Poster
- Document Year:
- 2023
- Event:
- Presenters:
- Akash Gupta
- Categories:
- Log in to post comments
Lack of audio-video synchronization is a common problem during television broadcasts and video conferencing, leading to an unsatisfactory viewing experience. A widely accepted paradigm is to create an error detection mechanism that identifies the cases when audio is leading or lagging. We propose ModEFormer, which independently extracts audio and video embeddings using modality-specific transformers. Different from the other transformer-based approaches, ModEFormer preserves the modality of the input streams which allows us to use a larger batch size with more negative audio samples for contrastive learning. Further, we propose a trade-off between the number of negative samples and number of unique samples in a batch to significantly exceed the performance of previous methods. Experimental results show that ModEFormer achieves state-of-the-art performance, 94.5% for LRS2 and 90.9% for LRS3. Finally, we demonstrate how ModEFormer can be used for offset detection for test clips.