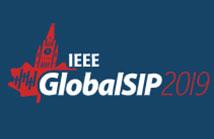
The 7th IEEE Global Conference on Signal and Information Processing (GlobalSIP) focuses on signal and information processing with an emphasis on up-and-coming signal processing themes. The conference features world-class plenary speeches, distinguished symposium talks, tutorials, exhibits, oral and poster sessions, and panels. GlobalSIP is comprised of co-located General Symposium and symposia selected based on responses to the call-for-symposia proposals.
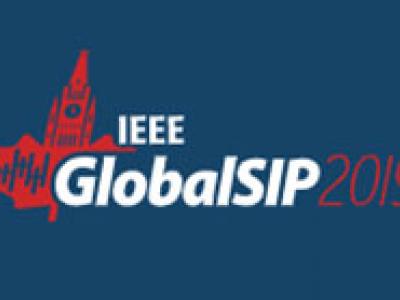
- Read more about Joint Subchannel and Power Allocation for Cognitive NOMA Systems with Imperfect CSI
- 1 comment
- Log in to post comments
In this paper, a robust power allocation and subchannel assignment algorithm was proposed to maximize the total EE
of SUs for cognitive NOMA systems under taking channel uncertainties and diverse QoS requirements of users into account. The RAA problem was formulated into a non-convex mixed-integer fractional programming problem with the outage probabilities of users and thus difficult to solve. Based on Gaussian CSI error models, we transformed the robust rate constraint and the robust interference power constraint into the convex constraints. By slacking the integer subchannel allocation factor into a continuous variable, the original problem was converted into a convex problem by using the subtractive-form auxiliary variable. Based on the Lagrangian dual approach and the subgradient updating methods, the closed-form solutions were obtained. The effectiveness of the proposed algorithm was verified by comparing it with the existing algorithms.
- Categories:
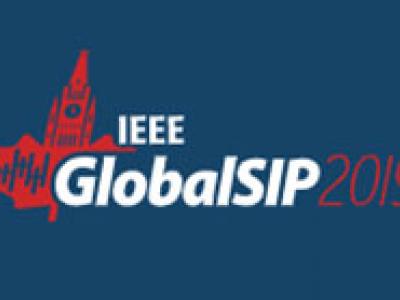
- Read more about Stochastic Tucker-Decomposed Recurrent Neural Networks for Forecasting
- Log in to post comments
The growing edge computing paradigm, notably the vision of the internet-of-things (IoT), calls for a new epitome of lightweight algorithms. Currently, the most successful models that learn from temporal data, which is prevalent in IoT applications, stem from the field of deep learning. However, these models evince extended training times and heavy resource requirements, prohibiting training in constrained environments. To address these concerns, we employ deep stochastic neural networks from the reservoir computing paradigm.
- Categories: