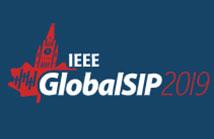
The 7th IEEE Global Conference on Signal and Information Processing (GlobalSIP) focuses on signal and information processing with an emphasis on up-and-coming signal processing themes. The conference features world-class plenary speeches, distinguished symposium talks, tutorials, exhibits, oral and poster sessions, and panels. GlobalSIP is comprised of co-located General Symposium and symposia selected based on responses to the call-for-symposia proposals.
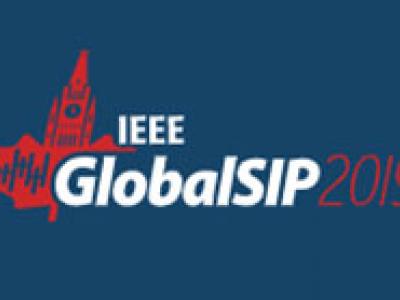
- Read more about Compressive Super-Pixel LiDAR for High-Framerate 3D Depth Imaging
- Log in to post comments
We propose a new sampling and reconstruction framework for full frame depth imaging using synchronised, programmable laser diode and photon detector arrays. By adopting a measurement scheme that probes the environment with sparse, pseudo-random patterns, our method enables eyesafe LiDAR operation, while guaranteeing fast reconstruction of
- Categories:
- Read more about Integrated Power and D2D Communications Simulator for Future Power Systems
- Log in to post comments
- Categories:
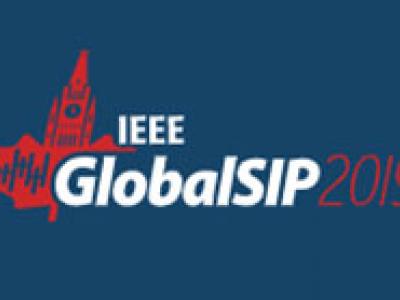
- Read more about Deep Learning Based Mass Detection in Mammograms
- Log in to post comments
Mammogram is the primary imaging technique for breast cancer screening, the leading type of cancer in women worldwide. While the clinical effectiveness of mammogram has been well demonstrated, the mammographic characteristics of breast masses are quite complex. As a result, radiologists certified for reading mammography are lacking, which limits the accessibility of mammography for more population. In this paper, we propose a Computer Aided Detection (CADe) method to automatically detect masses in mammography.
- Categories:
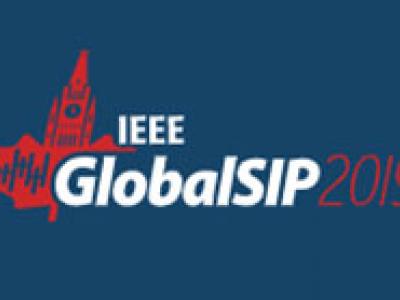
- Read more about AMA: An Open-source Amplitude Modulation Analysis Toolkit for Signal Processing Applications
- Log in to post comments
For their analysis with conventional signal processing tools, non-stationary signals are assumed to be stationary (or at least wide-sense stationary) in short intervals. While this approach allows them to be studied, it disregards the temporal evolution of their statistics. As such, to analyze this type of signals, it is desirable to use a representation that registers and characterizes the temporal changes in the frequency content of the signals, as these changes may occur in single or multiple periodic ways.
globalsip_2019.pdf

- Categories:
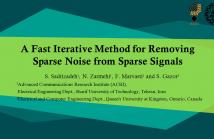
- Read more about A Fast Iterative Method for Removing Sparse Noise from Sparse Signals
- Log in to post comments
Reconstructing a signal corrupted by impulsive noise is of high importance in several applications, including impulsive noise removal from images, audios and videos, and separating texts from images. Investigating this problem, in this paper we propose a new method to reconstruct a noise-corrupted signal where both signal and noise are sparse but in different domains. We apply our algorithm for impulsive noise (Salt-and-Pepper Noise (SPN) and Random-Valued Impulsive Noise (RVIN) removal from images and compare our results with other notable algorithms in the literature.
- Categories:
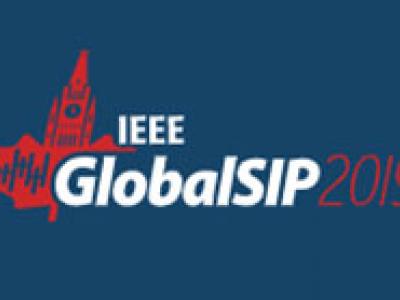
- Read more about Statistical Analysis of Antenna Array Systems with Perturbations in Phase, Gain and Element Positions
- Log in to post comments
In this paper, we statistically analyze the effect
of hardware impairments on power pattern of antenna array
systems. We consider a linear array and formulate the stochastic
beam pattern as a function of variations in phase, gain and
element positions. By deriving a closed-form expression for the
variance of the power pattern, we express how the performance
of antenna array can be degraded in each angle, allowing for
investigation of the role of each parameter in the final power
- Categories:
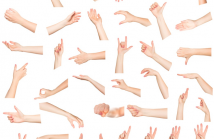
- Read more about SURFACE EMG-BASED HAND GESTURE RECOGNITION VIA DILATED CONVOLUTIONAL NEURAL NETWORKS
- 1 comment
- Log in to post comments
The recent evolution of Artificial Intelligence (AI) and deep learning models coupled with advancements of assistive robotic systems have shown great potential in significantly improving myoelectric control of prosthetic devices. In this regard, the paper proposes a novel deep-learning-based architecture for processing surface Electromyography (sEMG) signals to classify and recognize upper-limb hand gestures via incorporation of dilated causal convolutions.
- Categories:
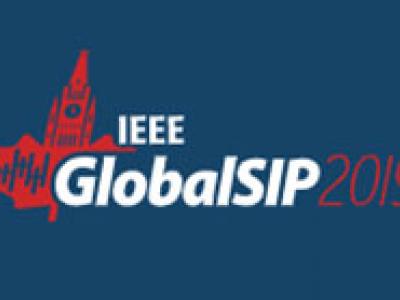
- Read more about GMM-UBM based Person Verification using footfall signatures for Smart Home Applications
- Log in to post comments
In this paper, we propose a novel person verification system based on footfall signatures using Gaussian Mixture Model-Universal Background Model (GMM-UBM). Ground vibration generated by footfall of an individual is used as a biometric modality. We conduct extensive experiments to compare the proposed technique with various baselines of footfall based person verification. The system is evaluated on an indigenous dataset containing 7750 footfall events of twenty subjects.
- Categories:
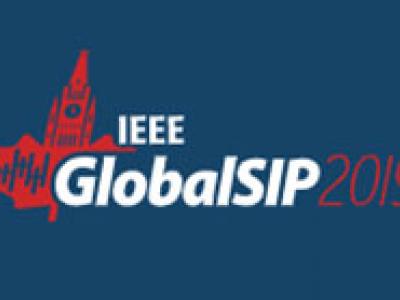
- Read more about Optimized Polarization Filtering Based Self-Interference Cancellation Scheme for Full-Duplex Communication
- Log in to post comments
In this paper, we propose an optimized polarization filtering based digital self-interference (SI) cancellation (OPC) scheme for full-duplex communication. The proposed OPC scheme utilizes polarization to increase the distinction between the desired signal and the SI and maximizes the signal to interference plus noise ratio (SINR) of the output signal.
- Categories:
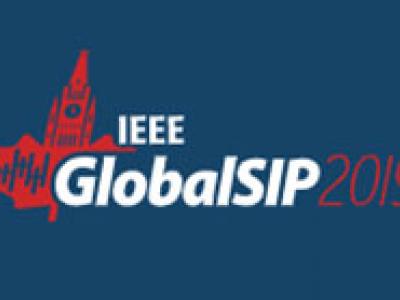
- Read more about Beam Alignment-Based mmWave Spectrum Sensing in Cognitive Vehicular Networks
- Log in to post comments
Millimeter wave (mmWave) communication is a promising technology to alleviate the shortage of spectrum resources in vehicular networks. To use mmWave spectrum resources more efficiently, in this paper we propose a novel beam alignment-based vehicular mmWave spectrum sensing model and algorithm. We first establish the spectrum sensing model on the basis of characteristics of mmWave signals and then derive the test statistics.
- Categories: