Documents
Poster
Feature Selection for Multi-labeled Variables via Dependency Maximization
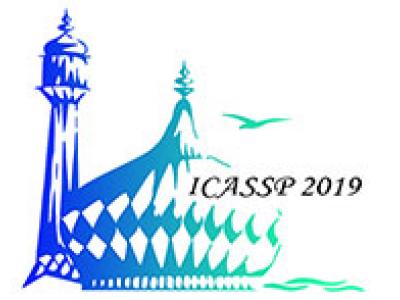
- Citation Author(s):
- Submitted by:
- Salimeh Yasaei Sekeh
- Last updated:
- 9 May 2019 - 9:36am
- Document Type:
- Poster
- Document Year:
- 2019
- Event:
- Presenters:
- Alfred O. Hero
- Paper Code:
- 4065
- Categories:
- Log in to post comments
Feature selection and reducing the dimensionality of data is an essential step in data analysis. In this work, we propose a new criterion for feature selection that is formulated as conditional information between features given the labeled variable. Instead of using the standard mutual information measure based on Kullback-Leibler divergence, we use our proposed criterion to filter out redundant features for the purpose of multiclass classification. This approach results in an efficient and fast non-parametric implementation of feature selection as it can be directly estimated using a geometric measure of dependency, the global Friedman-Rafsky (FR) multivariate run test statistic constructed by a global minimal spanning tree (MST). We demonstrate the advantages of our proposed feature selection approach through simulation. In addition, the proposed feature selection method is applied to the MNIST data set.