Documents
Presentation Slides
Mockingjay: Unsupervised Speech Representation Learning with Deep Bidirectional Transformer Encoders
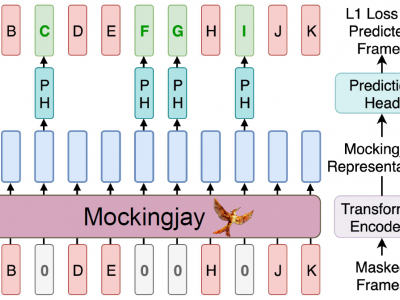
- Citation Author(s):
- Submitted by:
- Andy T. Liu
- Last updated:
- 15 May 2020 - 10:18pm
- Document Type:
- Presentation Slides
- Document Year:
- 2020
- Event:
- Presenters:
- Andy T. Liu
- Paper Code:
- SPE-L13.2
- Categories:
- Keywords:
- Log in to post comments
We present Mockingjay as a new speech representation learning approach, where bidirectional Transformer encoders are pre-trained on a large amount of unlabeled speech. Previous speech representation methods learn through conditioning on past frames and predicting information about future frames. Whereas Mockingjay is designed to predict the current frame through jointly conditioning on both past and future contexts. The Mockingjay representation improves performance for a wide range of downstream tasks, including phoneme classification, speaker recognition, and sentiment classification on spoken content, while outperforming other approaches. Mockingjay is empirically powerful and can be fine-tuned with downstream models, with only 2 epochs we further improve performance dramatically. In a low resource setting with only 0.1% of labeled data, we outperform the result of Mel-features that uses all 100% labeled data.