Documents
Poster
CFPNET: CHANNEL-WISE FEATURE PYRAMID FOR REAL-TIME SEMANTIC SEGMENTATION
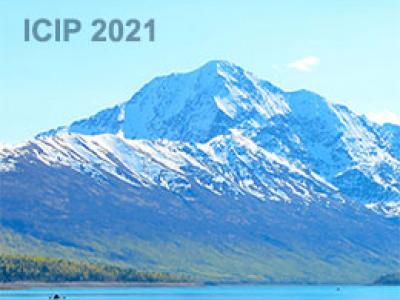
- Citation Author(s):
- Submitted by:
- Ange Lou
- Last updated:
- 23 September 2021 - 4:41pm
- Document Type:
- Poster
- Document Year:
- 2021
- Event:
- Presenters:
- Ange Lou
- Paper Code:
- 2937
- Categories:
- Log in to post comments
Real-time semantic segmentation is playing a more important role in computer vision, due to the growing demand for mobile devices and autonomous driving. Therefore, it is very important to achieve a good trade-off among performance, model size and inference speed. In this paper, we propose a Channel-wise Feature Pyramid (CFP) module to balance those factors. Based on the CFP module, we built CFPNet for real-time semantic segmentation which applied a series of dilated convolution channels to extract effective features. Experiments on Cityscapes and CamVid datasets show that the proposed CFPNet achieves an effective combination of those factors. For the Cityscapes test dataset, CFPNet achieves 70.1% class-wise mIoU with only 0.55 million parameters and 2.5 MB memory. The inference speed can reach 30 FPS on a single RTX 2080Ti GPU with a 1024x2048-pixel image.