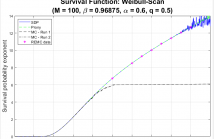
- Read more about New Results on the Weibull Distribution and Weibull Sums, with Application to Radar Sea Clutter
- Log in to post comments
This work covers and combines three themes concerning the Weibull distribution in the heavy-tailed region, as is relevant to sea clutter: (i) Characterizing the Weibull distribution as a compound clutter model and deriving a computationally tractable form for its implied texture distribution. (ii) Computing the distribution of a positively weighted sum of independent identically distributed (iid) Weibull random variables -- facilitated by the compound formulation, with emphasis on the tail.
- Categories:
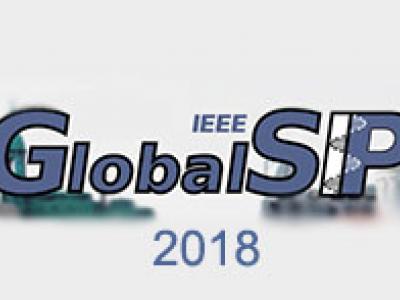
- Read more about Efficient RFI detection in radio astronomy based on Compressive Statistical Sensing
- Log in to post comments
In this paper, we present an efficient method for radio frequency interference (RFI) detection based on cyclic spectrum analysis that relies on compressive statistical sensing to estimate the cyclic spectrum from sub-Nyquist data. We refer to this method as compressive statistical sensing (CSS), since we utilize the statistical autocovariance matrix from the compressed data.
- Categories:
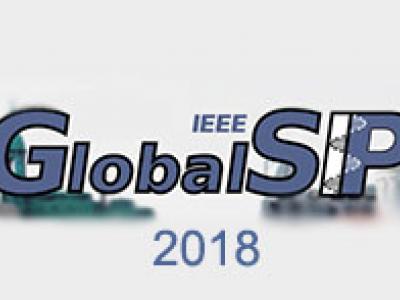
In the 3.5 GHz Citizens Broadband Radio Service (CBRS), 100 MHz of spectrum will be shared between commercial users and federal incumbents. Dynamic use of the band relies on a network of sensors dedicated to detecting the presence of federal incumbent signals and triggering protection mechanisms when necessary. This paper uses field-measured waveforms of incumbent signals in and adjacent to the band to evaluate the performance of matched-filter detectors for these sensors.
- Categories:
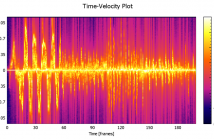
- Read more about Hidden Markov Model-based Gesture Recognition with FMCW Radar
- Log in to post comments
In this paper we present experimental results for the development
of a gesture recognition system using a 77 GHz FMCW
radar system. We measure the micro-Doppler signature of a
gesturing hand to construct an energy distribution in velocity
space over time. A gesturing hand is fundamentally a dynamical
system with unobservable “state” (i.e. the name of the gesture)
which determines the sequence of associated observable
velocity-energy distributions, so a Hidden Markov Model is
used to for gesture recognition, a more tailored approach than
- Categories: