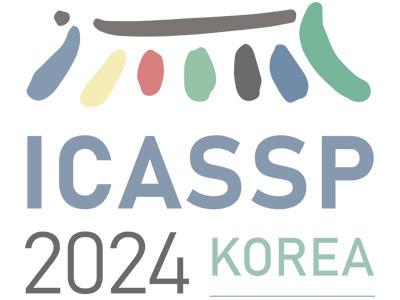
- Read more about dklement_dvbx_slides
- Log in to post comments
Bayesian HMM clustering of x-vector sequences (VBx) has become a widely adopted diarization baseline model in publications and challenges. It uses an HMM to model speaker turns, a generatively trained probabilistic linear discriminant analysis (PLDA) for speaker distribution modeling, and Bayesian inference to estimate the assignment of x-vectors to speakers. This paper presents a new framework for updating the VBx parameters using discriminative training, which directly optimizes a predefined loss.
- Categories:
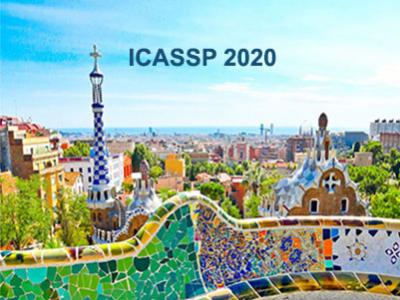
- Read more about Optimizing Bayesian HMM Based x-vector Clustering for theSecond DIHARD Speech Diarization Challenge
- Log in to post comments
- Categories:
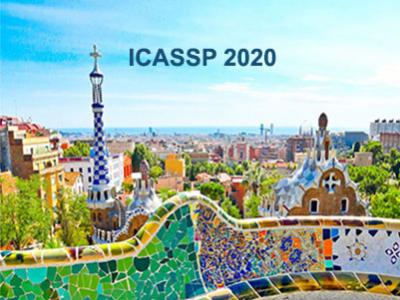
- Read more about Speaker Diarization with Session-level Speaker Embedding Refinement using Graph Neural Networks
- Log in to post comments
Deep speaker embedding models have been commonly used as a building block for speaker diarization systems; however, the speaker embedding model is usually trained according to a global loss defined on the training data, which could be sub-optimal for distinguishing speakers locally in a specific meeting session. In this work we present the first use of graph neural networks (GNNs) for the speaker diarization problem, utilizing a GNN to refine speaker embeddings locally using the structural information between speech segments inside each session.
- Categories: