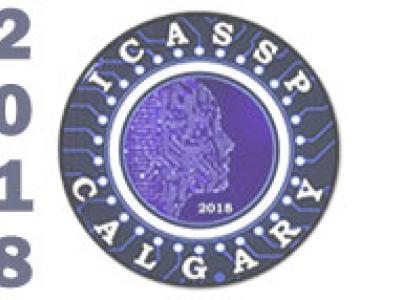
- Read more about ROBUST DETECTION OF JITTERED MULTIPLY REPEATING AUDIO EVENTS USING ITERATED TIME-WARPED ACF
- Log in to post comments
This paper proposes a novel approach for robustly detecting
multiply repeating audio events in monitoring recordings.
We consider the practically important case that
the sequence of inter onset intervals between subsequent events
is not constant but differs by some jitter. In such cases
classical approaches based on autocorrelation (ACF) are
of limited use. To overcome this problem we propose to use
ACF together with a variant of dynamic time warping. Combining
both techniques in an iterative algorithm, we obtain a
- Categories:
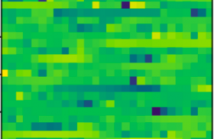
- Read more about Verbal Protest Recognition in Children with Autism
- Log in to post comments
Real-time detection of verbal protest (sensory overload-induced crying) in children with autism is a first step towards understanding the precursors of challenging behaviors associated with autism. Detection of verbal protest is useful for both autism researchers interested in exploring just-in-time intervention techniques and researchers interested in audio event detection in routine living environments.In this paper, we examine, adapt, and improve upon two techniques for verbal protest recognition and tailor them for children with autism spectrum disorder (ASD).
- Categories:
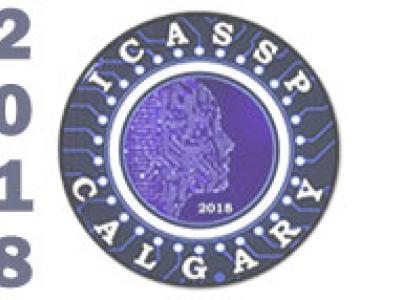
- Read more about AUTOMATIC BIRD VOCALIZATION IDENTIFICATION BASED ON FUSION OF SPECTRAL PATTERN AND TEXTURE FEATURES
- Log in to post comments
- Categories:
- Read more about Effect of Acoustic Conditions on Algorithms to Detect Parkinson’s Disease from Speech
- Log in to post comments
Automatic detection of Parkinson's disease (PD) from speech is a basic step towards computer-aided tools supporting the diagnosis and monitoring of the disease. Although several methods have been proposed, their applicability to real-world situations is still unclear. In particular, the effect of acoustic conditions is not well understood. In this paper, the effects on the accuracy of five different methods to detect PD from speech are evaluated.
- Categories:
- Read more about Online Learning of Time-Frequency Patterns
- Log in to post comments
We present an online method to learn recurring time-frequency patterns from spectrograms. Our method relies on a convolutive decomposition that estimates sequences of spectra into time-frequency patterns and their corresponding activation signals. This method processes one spectrogram at a time such that in comparison with a batch method, the computational cost is reduced proportionally to the number of considered spectrograms. We use a first-order stochastic gradient descent and show that a monotonically decreasing learning-rate works appropriately.
poster.pdf

- Categories:
- Read more about MULTI-VIEW REPRESENTATION LEARNING VIA GCCA FOR MULTIMODAL ANALYSIS OF PARKINSON'S DISEASE
- Log in to post comments
Information from different bio--signals such as speech, handwriting, and gait have been used to monitor the state of Parkinson's disease (PD) patients, however, all the multimodal bio--signals may not always be available. We propose a method based on multi-view representation learning via generalized canonical correlation analysis (GCCA) for learning a representation of features extracted from handwriting and gait that can be used as a complement to speech--based features. Three different problems are addressed: classification of PD patients vs.
- Categories:
Pages
- « first
- ‹ previous
- 1
- 2
- 3