- Read more about Unsupervised Accent Adaptation Through Masked Language Model Correction Of Discrete Self-Supervised Speech Units
- Log in to post comments
Self-supervised pre-trained speech models have strongly improved speech recognition, yet they are still sensitive to domain shifts and accented or atypical speech. Many of these models rely on quantisation or clustering to learn discrete acoustic units. We propose to correct the discovered discrete units for accented speech back to a standard pronunciation in an unsupervised manner. A masked language model is trained on discrete units from a standard accent and iteratively corrects an accented token sequence by masking unexpected cluster sequences and predicting their common variant.
- Categories:
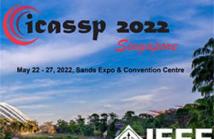
- Read more about Spell my name: keyword boosted speech recognition
- Log in to post comments
Recognition of uncommon words such as names and technical terminology is important to understanding conversations in context. However, the ability to recognise such words remains a challenge in modern automatic speech recognition (ASR) systems. In this paper, we propose a simple but powerful ASR decoding method that can better recognise these uncommon keywords, which in turn enables better readability of the results. The method boosts the probabilities of given keywords in a beam search based on acoustic model predictions. The method does not require any training in advance.
- Categories:
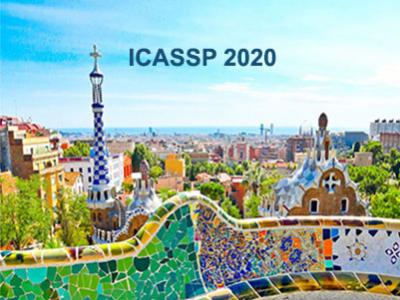
- Read more about PSEUDO LIKELIHOOD CORRECTION TECHNIQUE FOR LOW RESOURCE ACCENTED ASR
- Log in to post comments
With the availability of large data, ASRs perform well on native English but poorly for non-native English data.
Training nonnative ASRs or adapting a native English ASR is often limited by the availability of data, particularly for low resource scenarios. A typical HMM-DNN based ASR decoding requires pseudo-likelihood of states given an acoustic observation, which changes significantly from native to non-native speech due to accent variation.
- Categories:
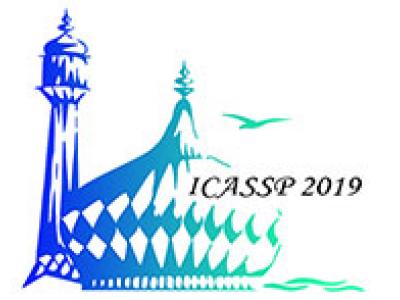
- Read more about BLHUC: BAYESIAN LEARNING OF HIDDEN UNIT CONTRIBUTIONS FOR DEEP NEURAL NETWORK SPEAKER ADAPTATION
- Log in to post comments
- Categories:
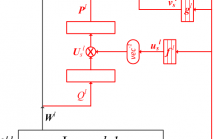
- Read more about Domain and speaker adaptation for Cortana Speech Recognition
- Log in to post comments
Voice assistant represents one of the most popular and important scenarios for speech recognition. In this paper, we propose two adaptation approaches to customize a multi-style well-trained acoustic model towards its subsidiary domain of Cortana assistant. First, we present anchor-based speaker adaptation by extracting the speaker information, i-vector or d-vector embeddings, from the anchor segments of `Hey Cortana'. The anchor embeddings are mapped to layer-wise parameters to control the transformations of both weight matrices and biases of multiple layers.
- Categories:
- Read more about Rapid Speaker Adaptation Based on D-code Extracted from BLSTM-RNN in LVCSR
- Log in to post comments
Recently, several fast speaker adaptation methods have been proposed for the hybrid DNN-HMM models based on the so called discriminative speaker codes (SC) and applied to unsupervised speaker adaptation in speech recognition. It has been demonstrated that the SC based methods are quite effective in adapting DNNs even when only a very small amount of adaptation data is available. However, in this way we have to estimate speaker code for new speakers by an updating process and obtain the final results through two-pass decoding.
- Categories: