- Read more about RESSCAL3D++: Joint Acquisition and Semantic Segmentation of 3D Point Clouds
- Log in to post comments
3D scene understanding is crucial for facilitating seamless interaction between digital devices and the physical world. Real-time capturing and processing of the 3D scene are essential for achieving this seamless integration. While existing approaches typically separate acquisition and processing for each frame, the advent of resolution-scalable 3D sensors offers an opportunity to overcome this paradigm and fully leverage the otherwise wasted acquisition time to initiate processing.
- Categories:
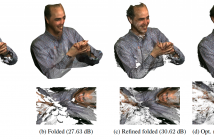
Existing techniques to compress point cloud attributes leverage either geometric or video-based compression tools. We explore a radically different approach inspired by recent advances in point cloud representation learning. Point clouds can be interpreted as 2D manifolds in 3D space. Specifically, we fold a 2D grid onto a point cloud and we map attributes from the point cloud onto the folded 2D grid using a novel optimized mapping method. This mapping results in an image, which opens a way to apply existing image processing techniques on point cloud attributes.
- Categories:
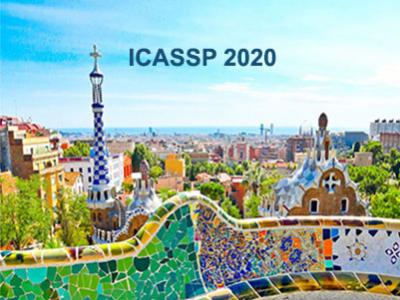
- Read more about COLOUR COMPRESSION OF PLENOPTIC POINT CLOUDS USING RAHT-KLT WITH PRIOR COLOUR CLUSTERING AND SPECULAR/DIFFUSE COMPONENT SEPARATION
- Log in to post comments
The recently introduced plenoptic point cloud representation marries a 3D point cloud with a light field. Instead of each point being associated with a single colour value, there can be multiple values to represent the colour at that point as perceived from different viewpoints. This representation was introduced together with a compression technique for the multi-view colour vectors, which is an extension of the RAHT method for point cloud attribute coding.
- Categories: