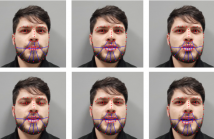
- Read more about DYNAMIC FACIAL FEATURES FOR INHERENTLY SAFER FACE RECOGNITION
- Log in to post comments
Among the many known type of intra-class variations, facial expressions are considered particularly challenging, as witnessed by the large number of methods that have been proposed to cope with them. The idea inspiring this work is that dynamic facial features (DFF) extracted from facial expressions while a sentence is pronounced, could possibly represent a salient and inherently safer biometric identifier, due to the greater difficulty in forging a time variable descriptor instead of a static one.
- Categories:
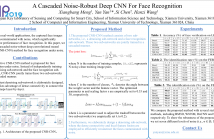
State-of-the-art face recognition methods have achieved ex- cellent performance on the clean datasets. However, in real- world applications, the captured face images are usually contaminated with noise, which significantly decreases the performance of these face recognition methods. In this pa- per, we propose a cascaded noise-robust deep convolutional neural network (CNR-CNN) method, consisting of two sub- networks, i.e., a denoising sub-network and a face recognition sub-network, for face recognition under noise.
- Categories:
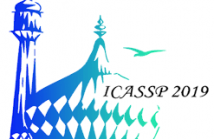
- Read more about Securing smartphone handwritten PIN codes with recurrent neural networks
- Log in to post comments
- Categories:
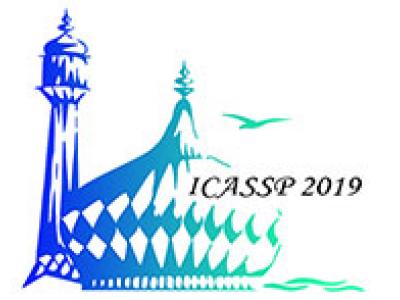
This paper proposes a group membership verification protocol preventing the curious but honest server from reconstructing the enrolled signatures and inferring the identity of querying clients. The protocol quantizes the signatures into discrete embeddings, making reconstruction difficult. It also aggregates multiple embeddings into representative values, impeding identification. Theoretical and experimental results show the trade-off between the security and error rates.
- Categories:
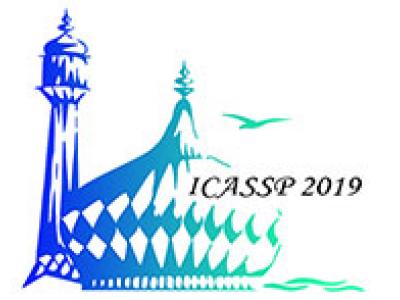
This paper proposes a group membership verification protocol preventing the curious but honest server from reconstructing the enrolled signatures and inferring the identity of querying clients. The protocol quantizes the signatures into discrete embeddings, making reconstruction difficult. It also aggregates multiple embeddings into representative values, impeding identification. Theoretical and experimental results show the trade-off between the security and error rates.
- Categories:
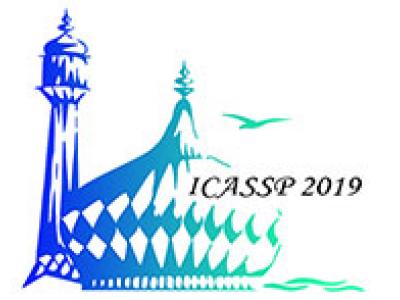
- Read more about CLEANING ADVERSARIAL PERTURBATIONS VIA RESIDUAL GENERATIVE NETWORK FOR FACE VERIFICATION
- Log in to post comments
Deep neural networks (DNNs) have recently achieved impressive performances on various applications. However, recent researches show that DNNs are vulnerable to adversarial perturbations injected into input samples. In this paper, we investigate a defense method for face verification: a deep residual generative network (ResGN) is learned to clean adversarial perturbations. We propose a novel training framework composed of ResGN, pre-trained VGG-Face network and FaceNet network.
poster苏玉莹.pdf

- Categories:
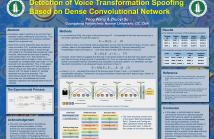
- Read more about DETECTION OF VOICE TRANSFORMATION SPOOFING BASED ON DENSE CONVOLUTIONAL NETWORK
- Log in to post comments
Nowadays, speech spoofing is so common that it presents a great challenge to social security. Thus, it is of great significance to recognize a spoofed speech from a genuine one. Most of the current researches have focused on voice conversion (VC), synthesis and recapture which mimic a target speaker to break through ASV systems by increased false acceptance rates. However, there exists another type of spoofing, voice transformation (VT), that transforms a speech signal without a target in order ‘not to be recognized’ by increased false reject rates. VT has received much less attention.
- Categories:
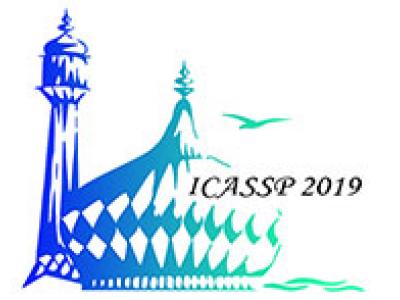
- Read more about ICASSP 2019 Poster for Paper #3198: PRIVACY-AWARE FEATURE EXTRACTION FOR GENDER DISCRIMINATION VERSUS SPEAKER IDENTIFICATION
- Log in to post comments
This paper introduces a deep neural network based feature extraction scheme that aims to improve the trade-off between utility and privacy in speaker classification tasks. In the proposed scenario we develop a feature representation that helps to maximize the performance of a gender classifier while minimizing additional speaker
- Categories:
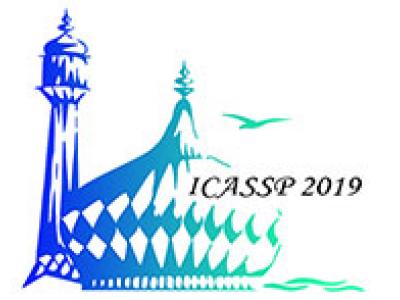
- Read more about Poster: Turning a vulnerability into an asset: Accelerating Facial Identification with Morphing
- Log in to post comments
In recent years, morphing of facial images has arisen as an important attack vector on biometric systems. Detection of morphed images has proven challenging for automated systems and human experts alike. Likewise, in recent years, the importance of efficient (fast) biometric identification has been emphasised by the rapid rise and growth of large-scale biometric systems around the world.
- Categories: