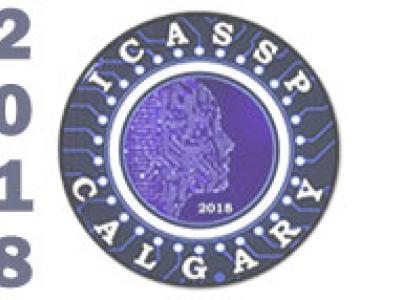
- Read more about GENERALISED DISCRIMINATIVE TRANSFORM VIA CURRICULUM LEARNING FOR SPEAKER RECOGNITION
- Log in to post comments
In this paper we introduce a speaker verification system deployed on mobile devices that can be used to personalise a keyword spotter. We describe a baseline DNN system that maps an utterance to a speaker embedding, which is used to measure speaker differences via cosine similarity. We then introduce an architectural modification which uses an LSTM system where the parameters are optimised via a curriculum learning procedure to reduce the detection error and improve its generalisability across various conditions.
- Categories:
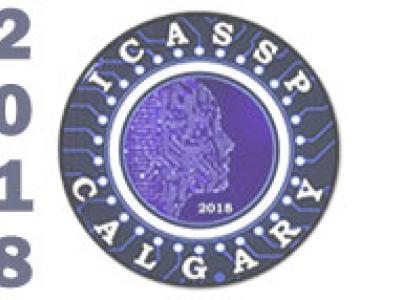
- Read more about A generative auditory model embedded neural network for speech processing
- Log in to post comments
Before the era of the neural network (NN), features extracted from auditory models have been applied to various speech applications and been demonstrated more robust against noise than conventional speech-processing features. What's the role of auditory models in the current NN era? Are they obsolete? To answer this question, we construct a NN with a generative auditory model embedded to process speech signals.
- Categories:
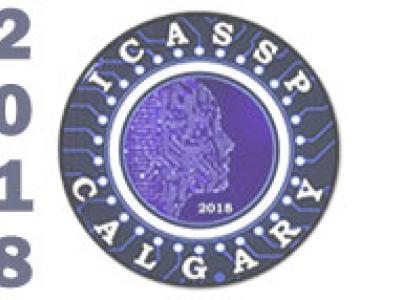
- Read more about A generative auditory model embedded neural network for speech processing
- Log in to post comments
Before the era of the neural network (NN), features extracted from auditory models have been applied to various speech applications and been demonstrated more robust against noise than conventional speech-processing features. What’s the role
of auditory models in the current NN era? Are they obsolete?
To answer this question, we construct a NN with a generative auditory model embedded to process speech signals. The
generative auditory model consists of two stages, the stage of spectrum estimation in the logarithmic-frequency axis by
- Categories:
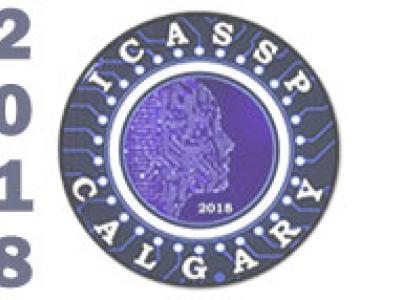
- Read more about DEEP FACTORIZATION FOR SPEECH SIGNAL
- Log in to post comments
Various informative factors mixed in speech signals, leading to great difficulty when decoding any of the factors. An intuitive idea is to factorize each speech frame into individual informative factors, though it turns out to be highly difficult. Recently, we found that speaker traits, which were assumed to be long-term distributional properties, are actually short-time patterns, and can be learned by a carefully designed deep neural network (DNN). This discovery motivated a cascade deep factorization (CDF) framework that will be presented in this paper.
- Categories:
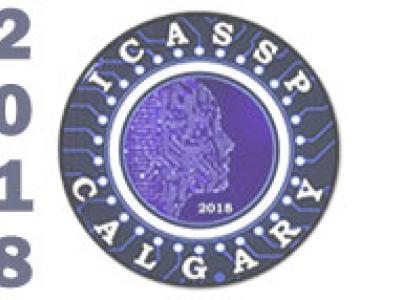
In recent studies, it has shown that speaker patterns can be learned from very short speech segments (e.g., 0.3 seconds) by a carefully designed convolutional & time-delay deep neural network (CT-DNN) model. By enforcing the model to discriminate the speakers in the training data, frame-level speaker features can be derived from the last hidden layer.
- Categories:
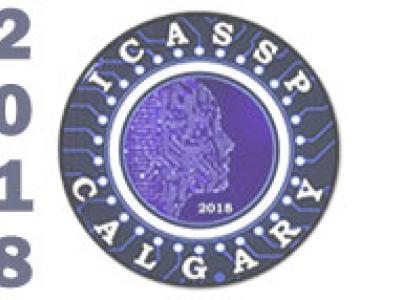
- Read more about SPEAKER-PHONETIC VECTOR ESTIMATION FOR SHORT DURATION SPEAKER VERIFICATION
- Log in to post comments
- Categories:
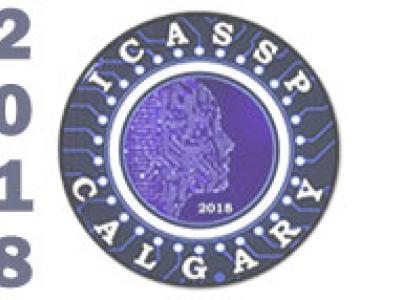
- Read more about Unsupervised Domain Adaptation for Gender-Aware PLDA Mixture Models
- Log in to post comments
- Categories:
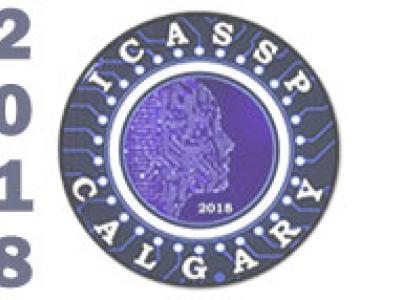
- Read more about A COMPLETE END-TO-END SPEAKER VERIFICATION SYSTEM USING DEEP NEURAL NETWORKS: FROM RAW SIGNALS TO VERIFICATION RESULT
- Log in to post comments
End-to-end systems using deep neural networks have been widely studied in the field of speaker verification. Raw audio signal processing has also been widely studied in the fields of automatic music tagging and speech recognition. However, as far as we know, end-to-end systems using raw audio signals have not been explored in speaker verification. In this paper, a complete end-to-end speaker verification system is proposed, which inputs raw audio signals and outputs the verification results. A pre-processing layer and the embedded speaker feature extraction models were mainly investigated.
- Categories:
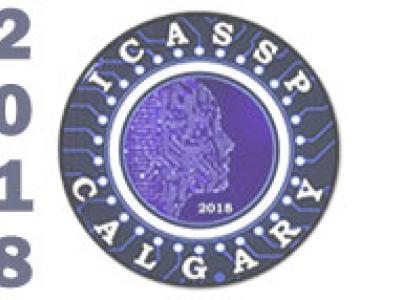
- Read more about END-TO-END DNN BASED SPEAKER RECOGNITION INSPIRED BY I-VECTOR AND PLDA
- Log in to post comments
- Categories:
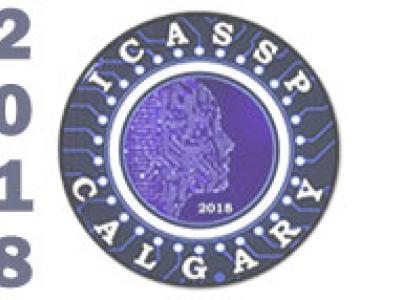
- Read more about Speaker-Phonetic Vector Estimation for Short Duration Speaker Verification
- Log in to post comments
Phonetic variability is one of the primary challenges in short duration speaker verification. This paper proposes a novel method that modifies the standard normal distribution prior in the total variability model to use a mixture of Gaussians as the prior distribution. The proposed speaker-phonetic vectors are then estimated from the posterior probability of latent variables, and each vector has a phonetic meaning.
- Categories: