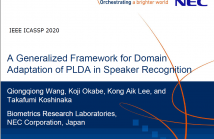
- Read more about A Generalized Framework for Domain Adaptation of PLDA in Speaker Recognition
- Log in to post comments
This paper proposes a generalized framework for domain adaptation of Probabilistic Linear Discriminant Analysis (PLDA) in speaker recognition. It not only includes several existing supervised and unsupervised domain adaptation methods but also makes possible more flexible usage of available data in different domains. In particular, we introduce here the two new techniques described below. (1) Correlation-alignment-based interpolation and (2) covariance regularization.
- Categories:
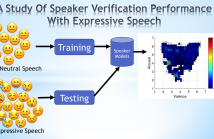
- Read more about A study of speaker verification performance with expressive speech
- Log in to post comments
Expressive speech introduces variations in the acoustic features affecting the performance of speech technology such as speaker verification systems. It is important to identify the range of emotions for which we can reliably estimate speaker verification tasks. This paper studies the performance of a speaker verification system as a function of emotions. Instead of categorical classes such as happiness or anger, which have important intra-class variability, we use the continuous attributes arousal, valence, and dominance which facili- tate the analysis.
- Categories:
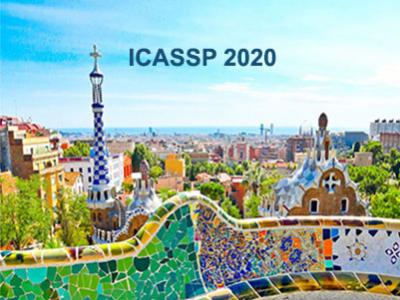
- Read more about COMBINING DEEP EMBEDDINGS OF ACOUSTIC AND ARTICULATORY FEATURES FOR SPEAKER IDENTIFICATION
- Log in to post comments
In this study, deep embedding of acoustic and articulatory features are combined for speaker identification. First, a convolutional neural network (CNN)-based universal background model (UBM) is constructed to generate acoustic feature (AC) embedding. In addition, as the articulatory features (AFs) represent some important phonological properties during speech production, a multilayer perceptron (MLP)-based AF embedding extraction model is also constructed for AF embedding extraction.
- Categories:
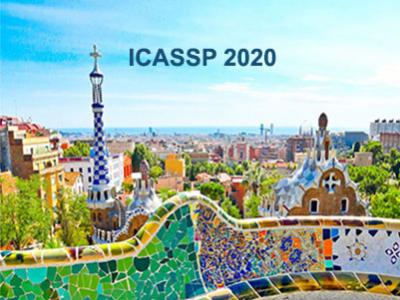
- Read more about Statistics Pooling Time Delay Neural Network Based on X-vector for Speaker Verification
- Log in to post comments
This paper aims to improve speaker embedding representation based on x-vector for extracting more detailed information for speaker verification. We propose a statistics pooling time delay neural network (TDNN), in which the TDNN structure integrates statistics pooling for each layer, to consider the variation of temporal context in frame-level transformation. The proposed feature vector, named as stats-vector, are compared with the baseline x-vector features on the VoxCeleb dataset and the Speakers in the Wild (SITW) dataset for speaker verification.
- Categories:
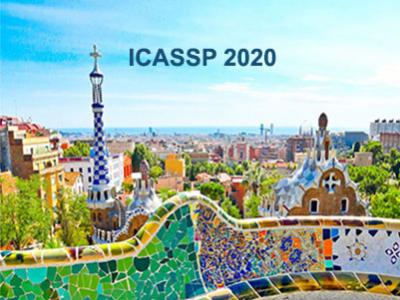
- Read more about BUT System for the Second DIHARD Speech Diarization Challenge
- Log in to post comments
This paper describes the winning systems developed by the BUT team for the four tracks of the Second DIHARD Speech Diarization Challenge. For tracks 1 and 2 the systems were mainly based on performing agglomerative hierarchical clustering (AHC) of x-vectors, followed by another x-vector clustering based on Bayes hidden Markov model and variational Bayes inference. We provide a comparison of the improvement given by each step and share the implementation of the core of the system.
- Categories:
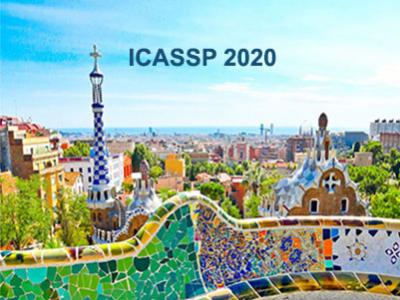
- Read more about MULTI-RESOLUTION MULTI-HEAD ATTENTION IN DEEP SPEAKER EMBEDDING
- 1 comment
- Log in to post comments
It is related to this paper: https://ieeexplore.ieee.org/document/9053217.
- Categories:
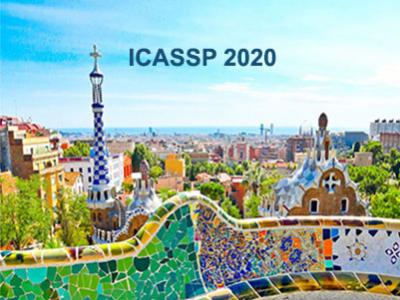
We address the problem of effectively handling overlapping speech in a diarization system. First, we detail a neural Long Short-Term Memory-based architecture for overlap detection. Secondly, detected overlap regions are exploited in conjunction with a frame-level speaker posterior matrix to make two-speaker assignments for overlapped frames in the resegmentation step. The overlap detection module achieves state-of-the-art performance on the AMI, DIHARD, and ETAPE corpora. We apply overlap-aware resegmentation on AMI, resulting in a 20% relative DER reduction over the baseline system.
- Categories:
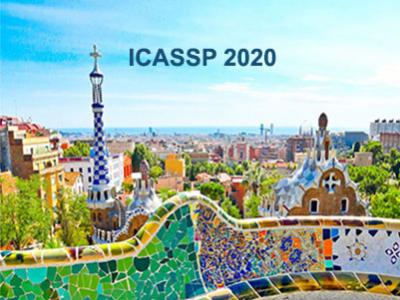
- Read more about Multi-level deep neural network adaptation for speaker verification using MMD and consistency regularization
- Log in to post comments
Adapting speaker verification (SV) systems to a new environ- ment is a very challenging task. Current adaptation methods in SV mainly focus on the backend, i.e, adaptation is carried out after the speaker embeddings have been created. In this paper, we present a DNN-based adaptation method using maximum mean discrepancy (MMD). Our method exploits two important aspects neglected by previous research.
- Categories:
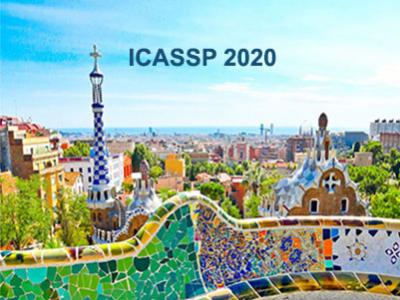
- Read more about Information Maximized Variational Domain Adversarial Learning for Speaker Verification
- Log in to post comments
Domain mismatch is a common problem in speaker ver- ification. This paper proposes an information-maximized variational domain adversarial neural network (InfoVDANN) to reduce domain mismatch by incorporating an InfoVAE into domain adversarial training (DAT). DAT aims to pro- duce speaker discriminative and domain-invariant features. The InfoVAE has two roles. First, it performs variational regularization on the learned features so that they follow a Gaussian distribution, which is essential for the standard PLDA backend.
- Categories:
- Read more about ICASSP2020 TEXT-INDEPENDENT SPEAKER VERIFICATION WITH ADVERSARIAL LEARNING ON SHORT UTTERANCES
- Log in to post comments
A text-independent speaker verification system suffers severe performance degradation under short utterance condition. To address the problem, in this paper, we propose an adversarially learned embedding mapping model that directly maps a short embedding to an enhanced embedding with increased discriminability. In particular, a Wasserstein GAN with a bunch of loss criteria are investigated. These loss functions have distinct optimization objectives and some of them are less favoured for the speaker verification research area.
- Categories: