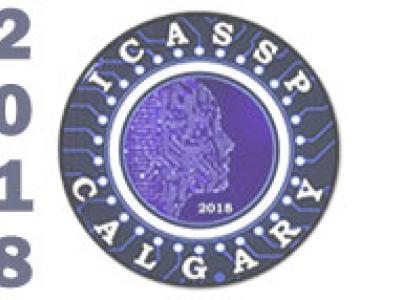
In this paper, we propose a new loss function called generalized end-to-end (GE2E) loss, which makes the training of speaker verification models more efficient than our previous tuple-based end-to-end (TE2E) loss function. Unlike TE2E, the GE2E loss function updates the network in a way that emphasizes examples that are difficult to verify at each step of the training process. Additionally, the GE2E loss does not require an initial stage of example selection.
- Categories:
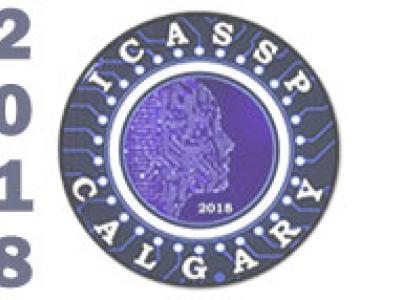
- Read more about A Novel Learnable Dictionary Encoding Layer for End-to-End Language Identification
- Log in to post comments
A novel learnable dictionary encoding layer is proposed in this paper for end-to-end language identification. It is inline with the conventional GMM i-vector approach both theoretically and practically. We imitate the mechanism of traditional GMM training and Supervector encoding procedure on the top of CNN. The proposed layer can accumulate high-order statistics from variable-length input sequence and generate an utterance level fixed-dimensional vector representation.
- Categories:
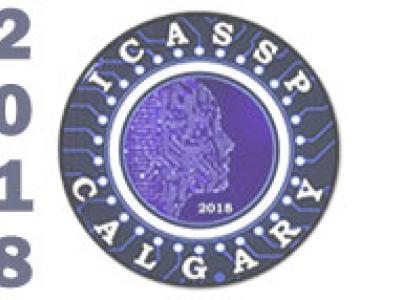
- Read more about Insights into End-to-End Learning Scheme for Language Identification
- Log in to post comments
A novel interpretable end-to-end learning scheme for language identification is proposed. It is in line with the classical GMM i-vector methods both theoretically and practically. In the end-to-end pipeline, a general encoding layer is employed on top of the front-end CNN, so that it can encode the variable-length input sequence into an utterance level vector automatically. After comparing with the state-of-the-art GMM i-vector methods, we give insights into CNN, and reveal its role and effect in the whole pipeline.
- Categories:
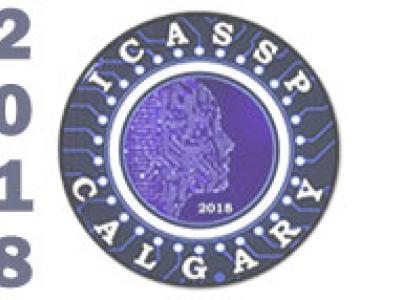
- Read more about DEREVERBERATION AND BEAMFORMING IN FAR-FIELD SPEAKER RECOGNITION
- Log in to post comments
This paper deals with far-field speaker recognition. On a corpus of NIST SRE 2010 data retransmitted in a real room with multiple microphones, we first demonstrate how room acoustics cause significant degradation of state-of-the-art i-vector based speaker recognition system. We then investigate several techniques to improve the performances ranging from probabilistic linear discriminant analysis (PLDA) re-training, through dereverberation, to beamforming.
- Categories:
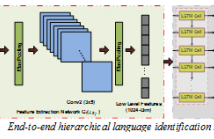
Recently, hierarchical language identification systems have shown significant improvement over single level systems in both closed and open set language identification tasks. However, developing such a system requires the features and classifier selection at each node in the hierarchical structure to be hand crafted. Motivated by the superior ability of end-to-end deep neural network architecture to jointly optimize the feature extraction and classification process, we propose a novel approach developing an end-to-end hierarchical language identification system.
- Categories:
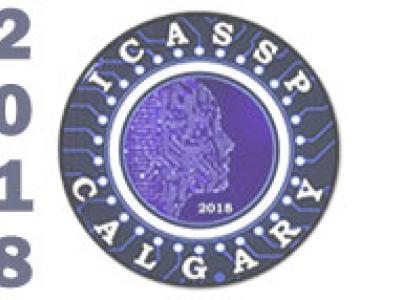
- Read more about MULTISTREAM DIARIZATION FUSION USING THE MINIMUM VARIANCE BAYESIAN INFORMATION CRITERION
- Log in to post comments
- Categories:
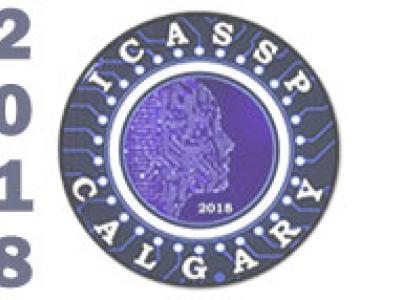
- Read more about Making Likelihood Ratios Digestible for Cross-Application Performance Assessment
- Log in to post comments
Performance estimation is crucial to the assessment of novel algorithms and systems. In detection error trade-off (DET) diagrams, discrimination performance is solely assessed targeting one application, where cross-application performance considers risks resulting from decisions, depending on application constraints. For the purpose of interchangeability of research results across different application constraints, we propose to augment DET curves by depicting systems regarding their support of security and convenience levels.
poster.pdf

- Categories:
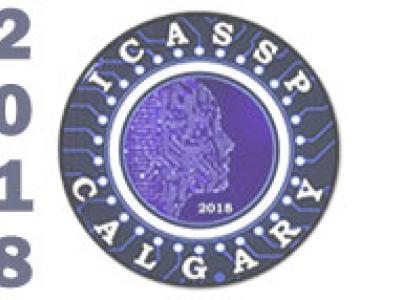
- Read more about Speaker Diarization with LSTM
- Log in to post comments
For many years, i-vector based audio embedding techniques were the dominant approach for speaker verification and speaker diarization applications. However, mirroring the rise of deep learning in various domains, neural network based audio embeddings, also known as d-vectors, have consistently demonstrated superior speaker verification performance. In this paper, we build on the success of d-vector based speaker verification systems to develop a new d-vector based approach to speaker diarization.
- Categories:
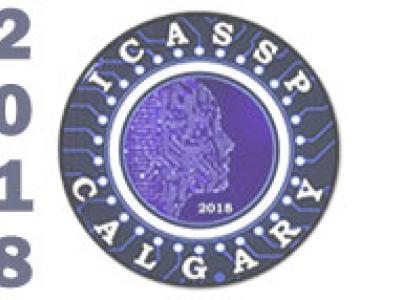
- Read more about ATTENTION-BASED MODELS FOR TEXT-DEPENDENT SPEAKER VERIFICATION
- Log in to post comments
Attention-based models have recently shown great performance on a range of tasks, such as speech recognition, machine translation, and image captioning due to their ability to summarize relevant information that expands through the entire length of an input sequence. In this paper, we analyze the usage of attention mechanisms to the problem of sequence summarization in our end-to-end text-dependent speaker recognition system. We explore different topologies and their variants of the attention layer, and compare different pooling methods on the attention weights.
- Categories:
- Read more about A new approach for robust replay spoof detection in ASV systems
- Log in to post comments
The objective of this paper is to extract robust features for
detecting replay spoof attacks on text-independent speaker
verification systems. In the case of replay attacks, prere-
corded utterance of the target speaker is played to the auto-
matic speaker verification system (ASV)to gain unauthorized
access. In such a scenario, the speech signal carries the char-
acteristics of the intermediate recording device as well. In the
proposed approach, the characteristics of the intermediate de-
- Categories: