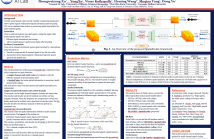
- Read more about SpatialCodec: Neural Spatial Speech Coding
- Log in to post comments
In this work, we address the challenge of encoding speech captured by a microphone array using deep learning techniques with the aim of preserving and accurately reconstructing crucial spatial cues embedded in multi-channel recordings. We propose a neural spatial audio coding framework that achieves a high compression ratio, leveraging single-channel neural sub-band codec and SpatialCodec.
- Categories:
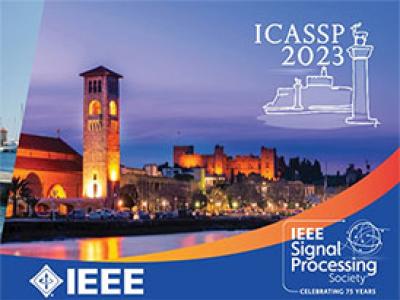
- Read more about SPEECH MODELING WITH A HIERARCHICAL TRANSFORMER DYNAMICAL VAE
- Log in to post comments
The dynamical variational autoencoders (DVAEs) are a family of latent-variable deep generative models that extends the VAE to model a sequence of observed data and a corresponding sequence of latent vectors. In almost all the DVAEs of the literature, the temporal dependencies within each sequence and across the two sequences are modeled with recurrent neural networks.
- Categories:
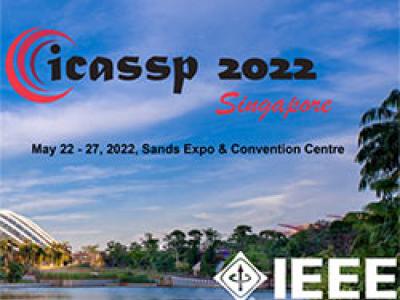
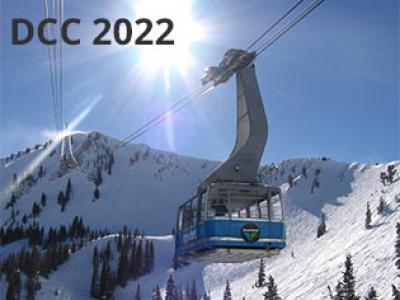
- Read more about A New Parametric Coding Method Combined Linear Microphone Array Topology
- Log in to post comments
The existing low bit rate multi-channel audio coding schemes basically depend on parametric model related to the psychoacoustics. On this basis, this paper aims at the linear microphone array, in which the redundancy between the array topology and signal is inventively exploited so that the coding efficiency is improved well. Compared with the classical schemes, the new parametric coding scheme proposed here effectively minimizes the amount of space parameters that need to be transmitted and greatly save the cost of transmission resources.
- Categories:
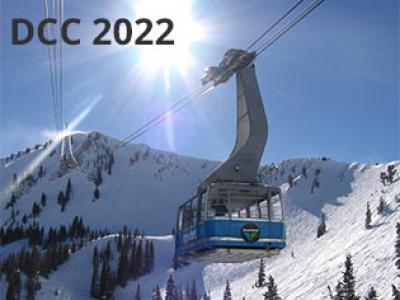
- Read more about DNN-based Multi-Channel Speech Coding Employing Sound Localization
- Log in to post comments
In this paper, a novel multi-channel speech coding method based on deep neural networks (DNN) employing sound localization called time difference of arrival (TDOA) is proposed. At the encoder, only the speech signals of two reference channels and estimated TDOAs are coded. At the decoder, a well-trained DNN that builds the relationship of the amplitude spectra between two reference channels and other channels is embedded into the decoder for recovering the amplitude spectra of other channel signals from two decoded reference signals.
- Categories:
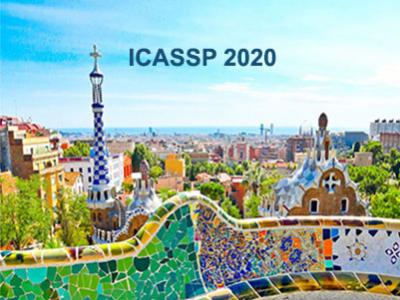
- Read more about Frame-based Overlapping Speech Detection using Convolutional Neural Networks
- Log in to post comments
Naturalistic speech recordings usually contain speech signals from multiple speakers. This phenomenon can degrade the performance of speech technologies due to the complexity of tracing and recognizing individual speakers. In this study, we investigate the detection of overlapping speech on segments as short as 25 ms using Convolutional Neural Networks. We evaluate the detection performance using different spectral features, and show that pyknogram features outperforms other commonly used speech features.
- Categories:
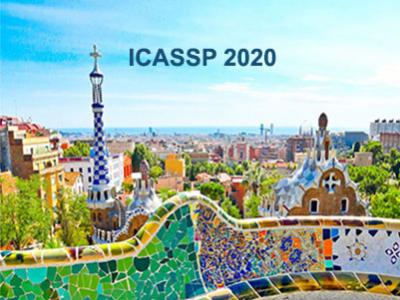
- Categories:
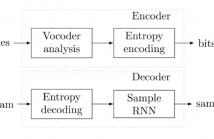
- Read more about HIGH-QUALITY SPEECH CODING WITH SAMPLE RNN
- Log in to post comments
We provide a speech coding scheme employing a generative model based on SampleRNN that, while operating at significantly lower bitrates, matches or surpasses the perceptual quality of state-of-the-art classic wide-band codecs. Moreover, it is demonstrated that the proposed scheme can provide a meaningful rate-distortion trade-off without retraining. We evaluate the proposed scheme in a series of listening tests and discuss limitations of the approach.
- Categories:
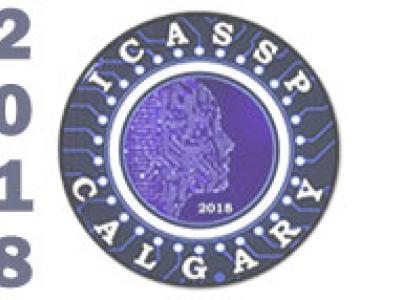
- Read more about GMM-BASED ITERATIVE ENTROPY CODING FOR SPECTRAL ENVELOPES OF SPEECH AND AUDIO
- 2 comments
- Log in to post comments
Spectral envelope modelling is a central part of speech and
audio codecs and is traditionally based on either vector quantization
or scalar quantization followed by entropy coding. To
bridge the coding performance of vector quantization with the
low complexity of the scalar case, we propose an iterative approach
for entropy coding the spectral envelope parameters.
For each parameter, a univariate probability distribution is derived
from a Gaussian mixture model of the joint distribution
Poster_GMM.pdf

- Categories: