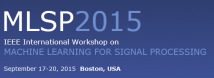
The 25th MLSP 2015 workshop in the series of workshops organized by the IEEE Signal Processing Society MLSP Technical Committee will take place in Boston USA and present the most recent and exciting advances in machine learning for signal processing through keynote talks, tutorials, as well as special and regular single-track sessions.
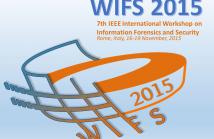
- Read more about Full-duplex vs. Half-duplex Secret-key Generation
- Log in to post comments
- Categories:
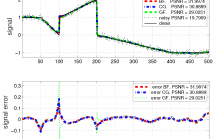
Graph-based spectral denoising is a low-pass filtering using the eigendecomposition of the graph Laplacian matrix of a noisy signal. Polynomial filtering avoids costly computation of the eigendecomposition by projections onto suitable Krylov subspaces. Polynomial filters can be based, e.g., on the bilateral and guided filters. We propose constructing accelerated polynomial filters by running flexible Krylov subspace based linear and eigenvalue solvers such as the Block Locally Optimal Preconditioned Conjugate Gradient (LOBPCG) method.
MLSP2015.pdf

- Categories:
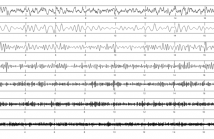
- Read more about Epileptic focus localization using EEG based on discrete wavelet transform through full-level decomposition
- Log in to post comments
Electroencephalogram (EEG) is a gold standard in epilepsy diagnosis and has been widely studied for epilepsy-related signal classification, such as seizure detection or focus localization. In the past few years, discrete wavelet transform (DWT) has been widely used to analyze epileptic EEG. However, one practical question unanswered is the optimal levels of wavelet decomposition. Deeper DWT can yield a more detailed depiction of signals but it requires substantially more computational time.
- Categories:
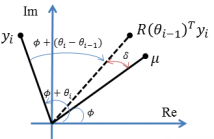
- Read more about A Sequential Bayesian Inference Framework for Blind Frequency Offset Estimation
- Log in to post comments
Precise estimation of synchronization parameters is essential for reliable
data detection in digital communications and phase errors can
result in significant performance degradation. The literature on estimation
of synchronization parameters, including the carrier frequency
offset, are based on approximations or heuristics because
the optimal estimation problem is analytically intractable for most
cases of interest. We develop an online Bayesian inference procedure
for blind estimation of the frequency offset, for arbitrary signal
- Categories: