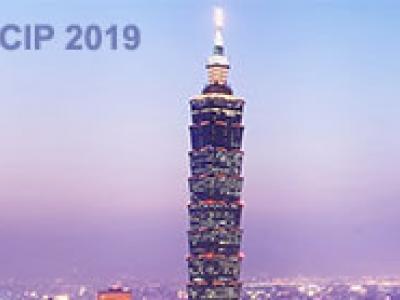
- Read more about Graph Regularization Network with Semantic Affinity for Weakly-supervised Temporal Action Localization
- Log in to post comments
This paper presents a novel deep architecture for weakly-supervised temporal action localization that predicts temporal boundaries with graph regularization. Our model not only generates segment-level action responses but also propagates segment-level responses to
neighborhood in a form of graph Laplacian regularization. Specifically, our approach consists of two sub-modules; a class activation
module to estimate the action score map over time through the action classifiers, and a graph regularization module to refine the
- Categories:
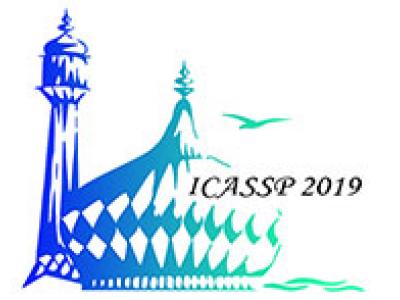
- Read more about EVALUATION OF NON-INTRUSIVE LOAD MONITORING ALGORITHMS FOR APPLIANCE-LEVEL ANOMALY DETECTION
- Log in to post comments
- Categories:
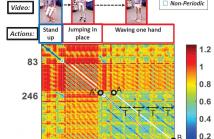
We present a solution to the problem of discovering all periodic
segments of a video and of estimating their period in
a completely unsupervised manner. These segments may be
located anywhere in the video, may differ in duration, speed,
period and may represent unseen motion patterns of any type
of objects (e.g., humans, animals, machines, etc). The proposed
method capitalizes on earlier research on the problem
of detecting common actions in videos, also known as commonality
detection or video co-segmentation. The proposed
- Categories:
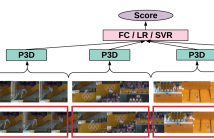
- Read more about S3D: Stacking Segmental P3D for Action Quality Assessment
- 1 comment
- Log in to post comments
Action quality assessment is crucial in areas of sports, surgery and assembly line where action skills can be evaluated. In this paper, we propose the Segment-based P3D-fused network S3D built-upon ED-TCN and push the performance on the UNLV-Dive dataset by a significant margin. We verify that segment-aware training performs better than full-video training which turns out to focus on the water spray. We show that temporal segmentation can be embedded with few efforts.
- Categories:
- Read more about When Harmonic Analysis Meets Machine Learning: Lipschitz Analysis of Deep Convolution Networks
- Log in to post comments
Deep neural networks have led to dramatic improvements in performance for many machine learning tasks, yet the mathematical reasons for this success remain largely unclear. In this talk we present recent developments in the mathematical framework of convolutive neural networks (CNN). In particular we discuss the scattering network of Mallat and how it relates to another problem in harmonic analysis, namely the phase retrieval problem. Then we discuss the general convolutive neural network from a theoretician point of view.
- Categories:
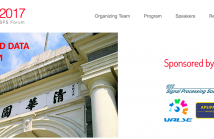
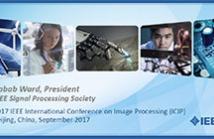
- Read more about ICIP 2017 - SPS Welcoming Remarks, Rabab Ward, IEEE SPS President
- Log in to post comments
IEEE Signal Processing Society welcoming remarks slides from IEEE President, Rabab Ward at ICIP 2017 on 18 September 2017 in Beijing, China.
Accompanying video can be fount on the Resource Center as well as IEEE SPS YouTube: https://www.youtube.com/watch?v=NRRzs0bB0a0.
- Categories:
- Read more about DenseNet for Dense Flow
- Log in to post comments
Efficient Large-Scale Video Understanding in The Wild
- Categories:
- Read more about CASCADED TEMPORAL SPATIAL FEATURES FOR VIDEO ACTION RECOGNITION
- Log in to post comments
Extracting spatial-temporal descriptors is a challenging task for video-based human action recognition. We decouple the 3D volume of video frames directly into a cascaded temporal spatial domain via a new convolutional architecture. The motivation behind this design is to achieve deep nonlinear feature representations with reduced network parameters. First, a 1D temporal network with shared parameters is first constructed to map the video sequences along the time axis into feature maps in temporal domain.
- Categories: