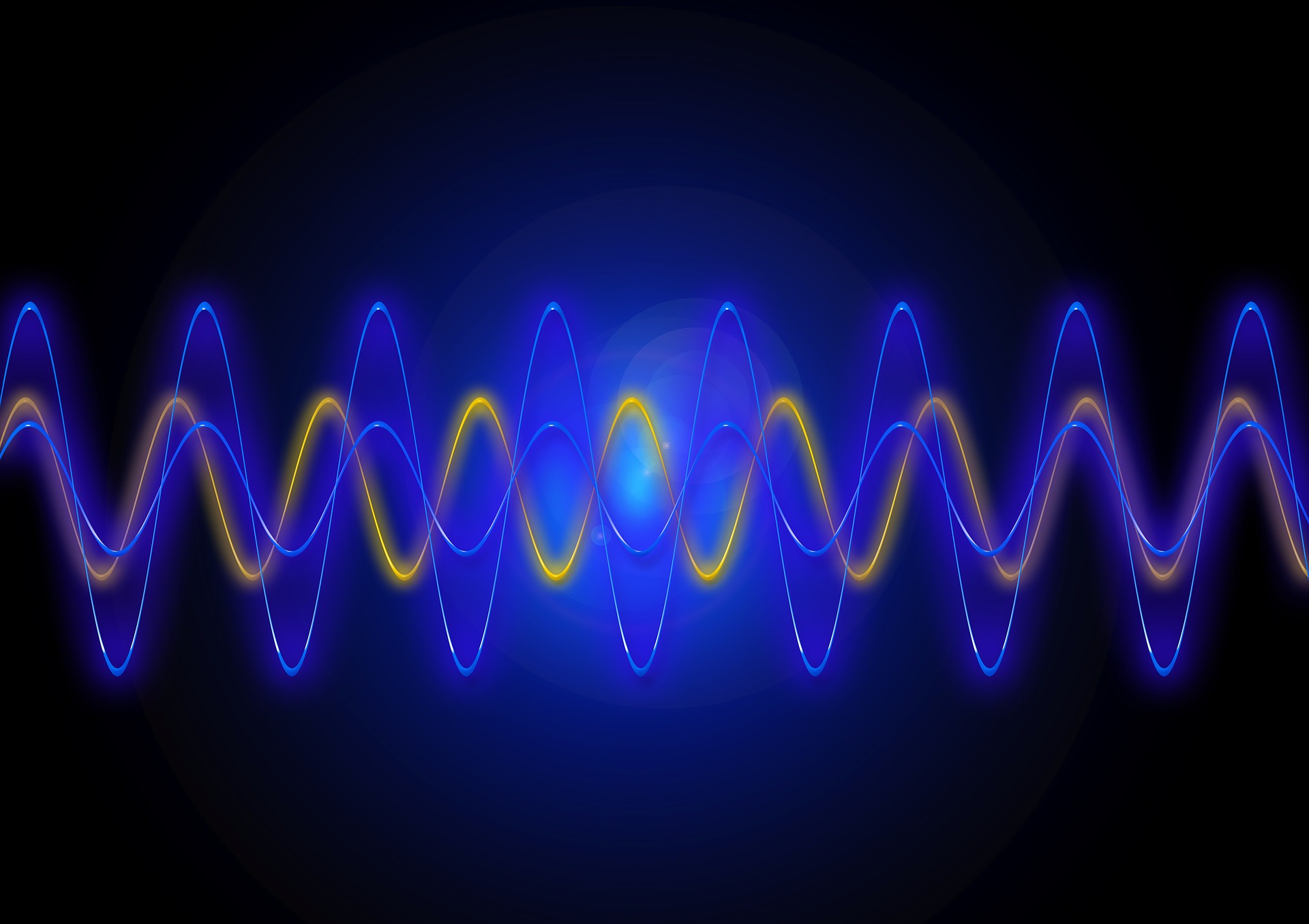
- Read more about Comparison of DCT and Autoencoder-based Features for DNN-HMM Multimodal Silent Speech Recognition
- Log in to post comments
poster-llc.pdf

- Categories:
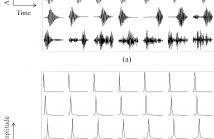
- Read more about FILTERBANK LEARNING USING CONVOLUTIONAL RESTRICTED BOLTZMANN MACHINE FOR SPEECH RECOGNITION
- Log in to post comments
Convolutional Restricted Boltzmann Machine (ConvRBM) as a model for speech signal is presented in this paper. We have
developed ConvRBM with sampling from noisy rectified linear units (NReLUs). ConvRBM is trained in an unsupervised way to model speech signal of arbitrary lengths. Weights of the model can represent an auditory-like filterbank. Our
poster.pdf

- Categories:
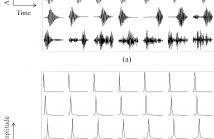
- Read more about FILTERBANK LEARNING USING CONVOLUTIONAL RESTRICTED BOLTZMANN MACHINE FOR SPEECH RECOGNITION
- Log in to post comments
Convolutional Restricted Boltzmann Machine (ConvRBM) as a model for speech signal is presented in this paper. We have
developed ConvRBM with sampling from noisy rectified linear units (NReLUs). ConvRBM is trained in an unsupervised way to model speech signal of arbitrary lengths. Weights of the model can represent an auditory-like filterbank. Our
poster.pdf

- Categories:
- Read more about Selection and Combination of Hypotheses for Dialectal Speech Recognition
- Log in to post comments
- Categories:
- Read more about Divergence estimation based on deep neural networks and its use for language identification
- Log in to post comments
In this paper, we propose a method to estimate statistical divergence between probability distributions by a DNN-based discriminative approach and its use for language identification tasks. Since statistical divergence is generally defined as a functional of two probability density functions, these density functions are usually represented in a parametric form. Then, if a mismatch exists between the assumed distribution and its true one, the obtained divergence becomes erroneous.
- Categories:
- Read more about ACCELERATING MULTI-USER LARGE VOCABULARY CONTINUOUS SPEECH RECOGNITION ON HETEROGENEOUS CPU-GPU PLATFORMS
- Log in to post comments
In our previous work, we developed a GPU-accelerated speech recognition engine optimized for faster than real time speech recognition on a heterogeneous CPU-GPU architecture. In this work, we focused on developing a scalable server-client architecture specifically optimized to simultaneously decode multiple users in real-time.
- Categories:
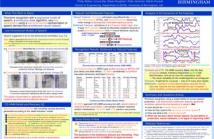
Recent advances in automatic speech recognition have used
large corpora and powerful computational resources to train
complex statistical models from high-dimensional features, to
attempt to capture all the variability found in natural speech.
Such models are difficult to interpret and may be fragile, and
contradict or ignore knowledge of human speech produc-
tion and perception. We report progress towards phoneme
recognition using a model of speech which employs very few
parameters and which is more faithful to the dynamics and
ICASSP2016.pdf

- Categories:
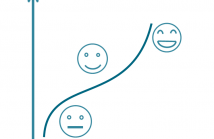
- Read more about Shaking and Speech-smile Vowels Classification: An Attempt at Amusement Arousal Estimation from Speech Signals
- Log in to post comments
In this paper, we present our work on speech-smile/shaking vowels classification. An efficient classification system would be a first step towards the estimation (from speech signals only) of amusement levels beyond smile, as indeed shaking vowels represent a transition from smile to laughter superimposed to speech. A database containing examples of both classes has been collected from acted and spontaneous speech corpora. An experimental study using several acoustic feature sets is presented here, and novel features are also proposed.
- Categories: