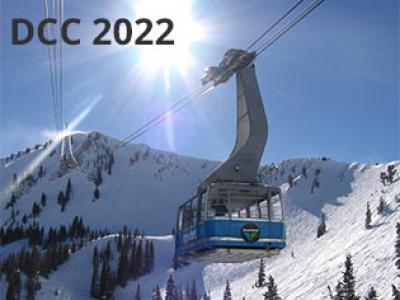
- Read more about A low-complexity destriping method for lossless compression of remote-sensing data
- Log in to post comments
- Categories:
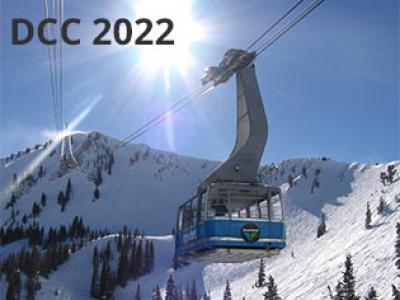
Existing methods for cross-component prediction focus on the chroma intra prediction but neglect the chroma prediction across multiple frames. In this paper, we propose a novel compression framework that leverages chroma frame sampling and inter-frame chroma prediction to address this problem. Specifically, chroma frame sampling discards the chroma components in inter-predicted frames to further reduce the bit consumption, whereas inter-frame chroma prediction recovers the discarded chroma components with optical flow estimation and post-training optimization for a guaranteed fidelity.
- Categories:
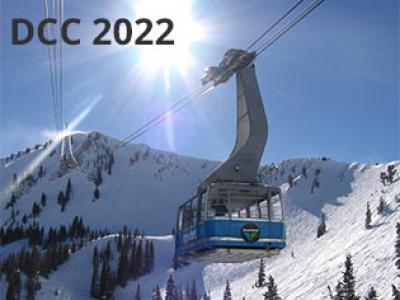
- Read more about Cross-component Sample Adaptive Offset
- Log in to post comments
This paper proposes one new in-loop filtering technique cross-component sample adaptive offset (CCSAO) for further coding efficiency improvement beyond Versatile Video Coding (VVC). The CCSAO reduces the sample distortion by 1) utilizing the strong correlation between luma and chroma components to classify the reconstructed samples into different categories and 2) deriving one offset for each category and adding the offset to the samples in the category. The offset of each category is properly derived at encoder and signaled to decoder.
- Categories:
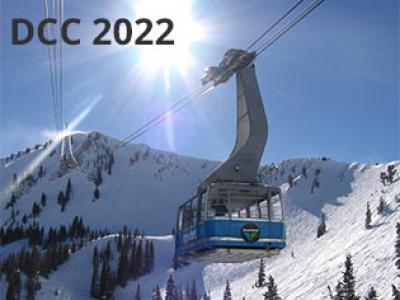
- Read more about An Improvement to Intra Block Copy in VVC with Reference Area Redefinition
- Log in to post comments
DCC2022_xu.pdf

- Categories:
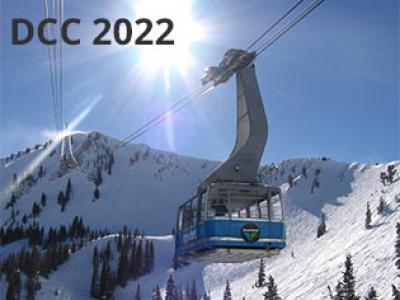
- Read more about Concurrent Encryption and Lossless Compression using Inversion Ranks
- Log in to post comments
dcc_202.pdf

- Categories:
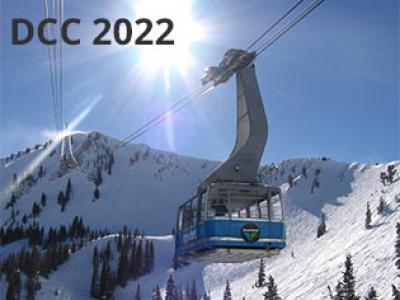
- Read more about Analysis of Lossless Compressors Applied to Integer and Floating-Point Astronomical Data
- 1 comment
- Log in to post comments
- Categories:
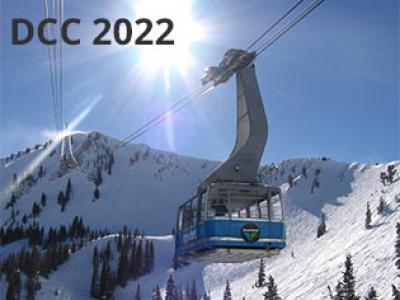
- Read more about Learning True Rate-Distortion-Optimization for End-To-End Image Compression
- Log in to post comments
- Categories:
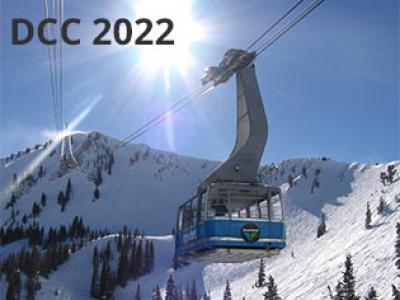
- Read more about Analysis on Compressed Domain: A Multi-Task Learning Approach
- 2 comments
- Log in to post comments
Image compression approaches based on deep learning have achieved remarkable success.
Existing studies mainly focus on human vision and machine analysis tasks taking reconstructed images as input.
- Categories:
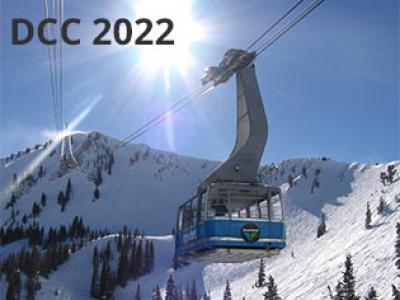
- Read more about Entropy Modeling via Gaussian Process Regression for Learned Image Compression
- Log in to post comments
- Categories: