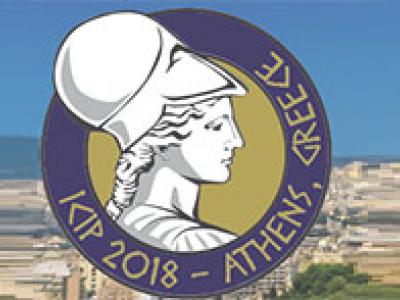
- Read more about REAL-TIME REGISTRATION OF RGB-D IMAGE PAIR FOR SEE-THROUGH SYSTEM
- Log in to post comments
This paper presents a dense method of real-time registration of RGB-D image pair. So far, we have proposed the “see-
through system”, in which multiple images acquired from RGB-D sensors are integrated to present images that is useful for confirming the shape or the positions of objects behind obstacles. However, errors of positional relation of sensors re-
- Categories:
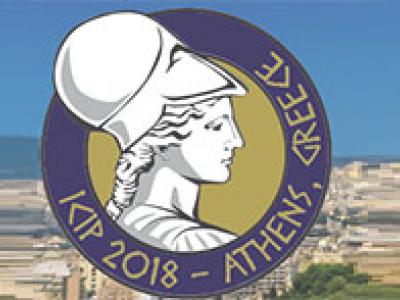
This paper presents an empirical Bayesian method to estimate regularisation parameters in imaging inverse problems. The method calibrates regularisation parameters directly from the observed data by maximum marginal likelihood estimation, and is useful for inverse problems that are convex. A main novelty is that maximum likelihood estimation is performed efficiently by using a stochastic proximal gradient algorithm that is driven by two proximal Markov chain Monte Carlo samplers, intimately combining modern optimisation and sampling techniques.
- Categories:
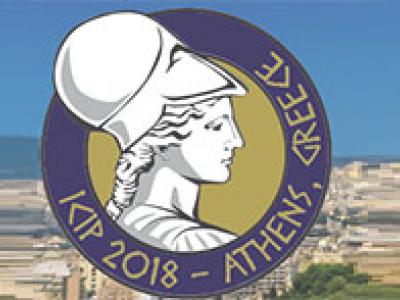
- Read more about BAYESIAN APPROACH FOR AUTOMATIC JOINT PARAMETER ESTIMATION IN 3D IMAGE RECONSTRUCTION FROM MULTI-FOCUS MICROSCOPE
- Log in to post comments
- Categories:
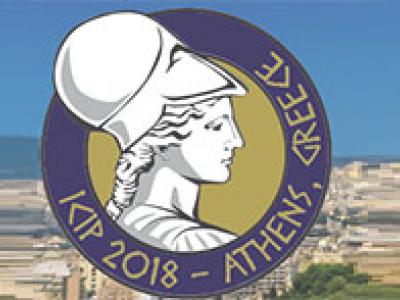
- Read more about ICIP18001
- Log in to post comments
Traditional methods for multiple object tracking usually consider features at image level and reason about simple space and time constraints. However, in this paper we propose a multiple object tracker based on LSTM network to learn temporally correlated features. Our tracker learns features on velocity, position and appearance aspects of the objects to improve tracking accuracy. In order to deal with occlusion problems, velocity model is also used to predict the lost detections for the occluded identities.
- Categories:
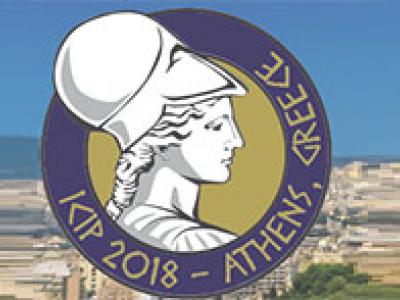
We propose a nonrigid registration method whose motion estimation is cast into a feature matching problem under the Log-Demons framework using Graph Wavelets. We investigate the Spectral Graph Wavelets (SGWs) to capture the shape features of the images. The SGWs are more adapted to learn the spatial and geometric organization of data with complex structures than the classical wavelets. Our experiments on T1 brain images and endomicroscopic images show that this method outperforms the existing nonrigid image registration techniques (i.e.
- Categories:
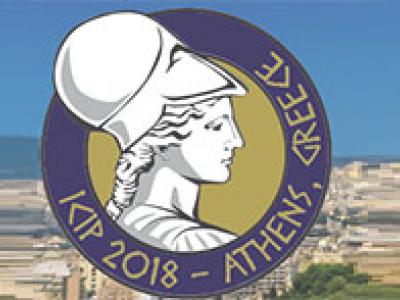
- Read more about Registration and Fusion of Multi-Spectral Images Using a Novel Edge Descriptor
- Log in to post comments
In this work we propose a fully end-to-end approach for
multi-spectral image registration and fusion. Our fusion
method combines images from different spectral channels
into a single fused image using approaches for low and high
frequency signals. A prerequisite of fusion is the geometric
alignment between the spectral bands, commonly referred to
as registration. Unfortunately, common methods for image
registration of a single spectral channel might prove inaccurate
on images from different modalities. For that end, we
icipPoster.pdf

- Categories:
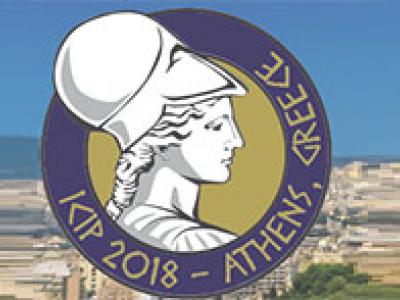
- Read more about Deep Multi-Spectral Registration Using Invariant Descriptor Learning
- Log in to post comments
In this work, we propose a deep-learning approach for aligning
cross-spectral images. Our approach utilizes a learned
descriptor invariant to different spectra. Multi-modal images
of the same scene capture different characteristics and therefore
their registration is challenging. To that end, we developed
a feature-based approach for registering visible (VIS)
to Near-Infra-Red (NIR) images. Our scheme detects corners
by Harris and matches them by a patch-metric learned
on top of a network trained using the CIFAR-10 dataset. As
- Categories:
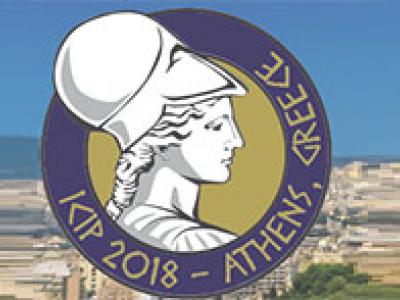
- Read more about GRAPH-BASED EARLY-FUSION FOR FLOOD DETECTION
- Log in to post comments
Flooding is one of the most harmful natural disasters, as it poses danger to both buildings and human lives. Therefore, it is fundamental to monitor these disasters to define prevention strategies and help authorities in damage control. With the wide use of portable devices (e.g., smartphones), there is an increase of the documentation and communication of flood events in social media. However, the use of these data in monitoring systems is not straightforward and depends on the creation of effective recognition strategies.
- Categories:
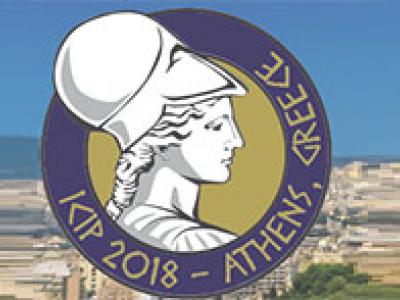
Unpaired image-to-image translation is a tricky task which aims at learning a mapping from one image collection to another image collection without any pair-labeled information. Recent works have proposed cycle-consistency assumption to deal with this task. However, the result is still unsatisfactory for geometric translation. To address this limitation, this paper proposes a novel method using shared deep space generative adversarial network (SDSGAN).
- Categories:
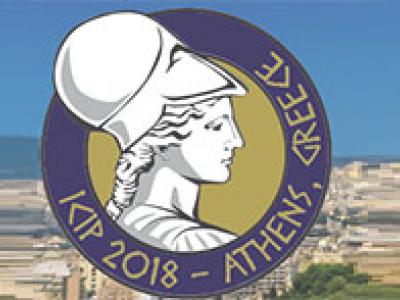
- Read more about Trained perceptual transform for quality assessment of high dynamic range images and video
- Log in to post comments
- Categories: