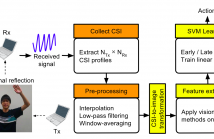
- Read more about WiFi Action Recognition via Vision based Methods
- Log in to post comments
Action recognition via WiFi has caught intense attention recently because of its ubiquity, low cost, and privacy- preserving. Observing Channel State Information (CSI, a fine-grained information computed from the received WiFi signal) resemblance to texture, we transform the received CSI into images, extract features with vision-based methods and train SVM classifiers for action recognition. Our experiments show that regarding CSI as images achieves an accuracy above 85%. Our contributions include:
- Categories:
- Read more about Fast Keypoint Detection in Video Sequences
- Log in to post comments
Several computer vision tasks exploit a succinct representation of the visual content in the form of sets of local features. Given an input image, feature extraction algorithms identify key-points and assign to each of them a descriptor, based on the characteristics of the surrounding visual content. Several tasks might require local features to be extracted from a video sequence, on a frame-by-frame basis.
- Categories:
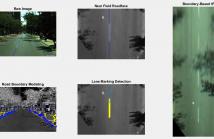
- Read more about Robust Lane Marking Detection using Boundary-Based Inverse Perspective Mapping
- 2 comments
- Log in to post comments
- Categories:
- Read more about SINGLE IMAGE BRIGHTENING VIA EXPOSURE FUSION
- Log in to post comments
- Categories:
- Read more about Learning Full-Range Affinity for Diffusion-based Saliency Detection
- Log in to post comments
- Categories:
- Read more about A NOVEL VIDEO-BASED SMOKE DETECTION METHOD BASED ON COLOR INVARIANTS
- Log in to post comments
In this paper, we address the issue of designing a smoke detector robust to illumination variations. Our contribution consists in resorting to color invariants as salient smoke features. More precisely, the proposed detector employs consecutively of an illumination invariant color representation, a photometric gain based background subtraction, a chrominance detection and a smoke identification based on two invariant color descriptors.
- Categories:
- Read more about Fall Detection in RGB-D Videos by Combining Shape and Motion Features
- Log in to post comments
- Categories:
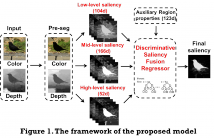
- Read more about Depth-aware Saliency Detection Using Discriminative Saliency Fusion
- Log in to post comments
Additional depth information from RGBD images is one of characteristics different from conventional 2D images. Saliency detection aims to detect the attractive objects to human viewers in an image. Generally, saliency cues from different features are measured and fused into a single saliency using a linear or experiential fusion formula. we introduce a multi-stage depth-aware saliency model to fuse multiple saliency maps in a discriminative method.
- Categories:
- Read more about CONTINUOUS ULTRASOUND BASED TONGUE MOVEMENT VIDEO SYNTHESIS FROM SPEECH
- Log in to post comments
The movement of tongue plays an important role in pronunciation. Visualizing the movement of tongue can improve speech intelligibility and also helps learning a second language. However, hardly any research has been investigated for this topic. In this paper, a framework to synthesize continuous ultrasound tongue movement video from speech is presented. Two different mapping methods are introduced as the most important parts of the framework. The objective evaluation and subjective opinions show that the Gaussian
- Categories: