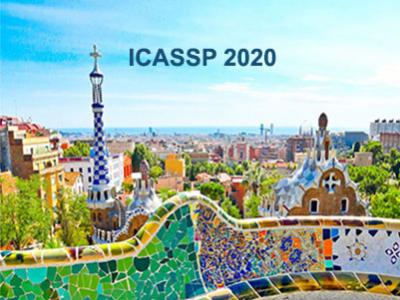
- Read more about DOA ESTIMATION IN SYSTEMS WITH NONLINEARITIES FOR MMWAVE COMMUNICATIONS
- 1 comment
- Log in to post comments
Accurate and efficient methods for Direction of Arrival (DOA) estimation play an important role in mmWave channel estimation methods. This estimation procedure can potentially be affected by the different RF and analog components in the communication system. Such components add an unknown, nonlinear distortion to the received signal. This work looks at addressing this problem of DOA estimation for a general case of a nonlinear distortion of the received signal. Two different scenarios for angle recovery are considered here: with the use of pilot symbols and without the use of pilots.
- Categories:
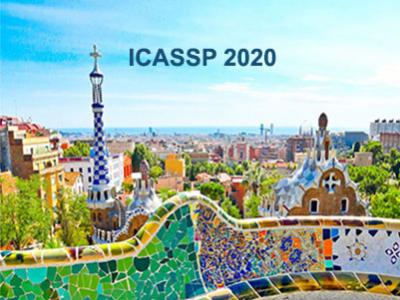
Arrays of closely-spaced antennas with mutual coupling have been considered recently with analogies to the hearing mechanism in small insects that exhibit excellent direction finding capabilities. We develop a model for a two-element array system that includes three distinct noise sources and a 4-port electrical network that couples the antennas to the measurement loads. The optimum coupling network that minimizes the Cramer-Rao bound (CRB) for angle of arrival (AOA) estimation is derived and a design method is presented to synthesize the network.
- Categories:
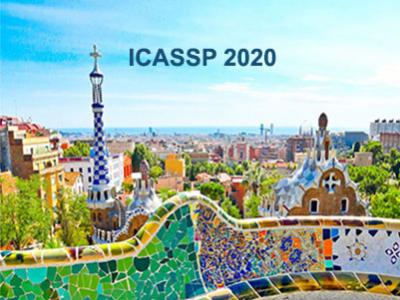
- Read more about Extended Object Tracking using Hierarchical Truncation Measurement Model with Automotive Radar
- 1 comment
- Log in to post comments
Motivated by real-world automotive radar measurements that are distributed around object (e.g., vehicles) edges with a certain volume, a novel hierarchical truncated Gaussian measurement model is proposed to resemble the underlying spatial distribution of radar measurements. With the proposed measurement model, a modified random matrix-based extended object tracking algorithm is developed to estimate both kinematic and extent states. In particular, a new state update step and an online bound estimation step are proposed with the introduction of pseudo measurements.
- Categories:
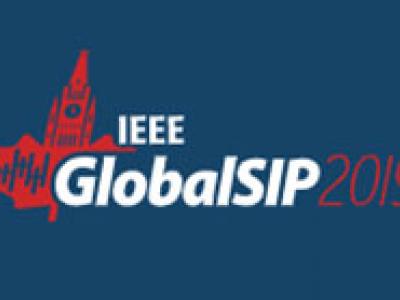
- Read more about Robust Direction of Arrival Estimation in the Presence of Array Faults using Snapshot Diversity
- Log in to post comments
- Categories:
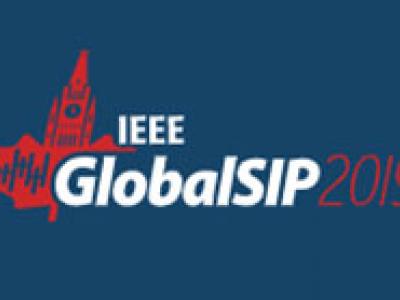
- Read more about Statistical Analysis of Antenna Array Systems with Perturbations in Phase, Gain and Element Positions
- Log in to post comments
In this paper, we statistically analyze the effect
of hardware impairments on power pattern of antenna array
systems. We consider a linear array and formulate the stochastic
beam pattern as a function of variations in phase, gain and
element positions. By deriving a closed-form expression for the
variance of the power pattern, we express how the performance
of antenna array can be degraded in each angle, allowing for
investigation of the role of each parameter in the final power
- Categories:
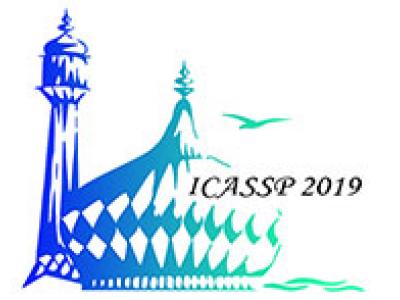
- Read more about Sample Space-Time Covariance Estimation
- Log in to post comments
Estimation errors are incurred when calculating the sample space-time covariance matrix. We formulate the variance of this estimator when operating on a finite sample set, compare it to known results, and demonstrate its precision in simulations. The variance of the estimation links directly to previously explored perturbation of the analytic eigenvalues and eigenspaces of a parahermitian cross-spectral density matrix when estimated from finite data.
icassp_2a.pdf

- Categories:
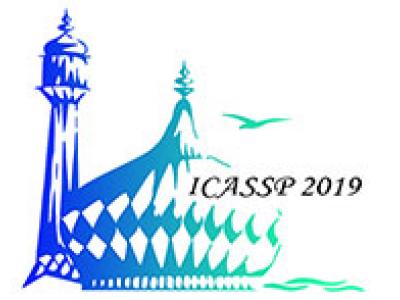
- Read more about Super-Resolution DOA Estimation for Arbitrary Array Geometries Using a Single Noisy Snapshot
- Log in to post comments
We address the problem of search-free DOA estimation from a single noisy snapshot for sensor arrays of arbitrary geometry, by extending a method of gridless super-resolution beam-forming to arbitrary arrays with noisy measurements. The primal atomic norm minimization problem is converted to a dual problem in which the periodic dual function is represented with a trigonometric polynomial using truncated Fourier series. The number of terms required for accurate representation depends linearly on the distance of the farthest sensor from a reference.
- Categories:
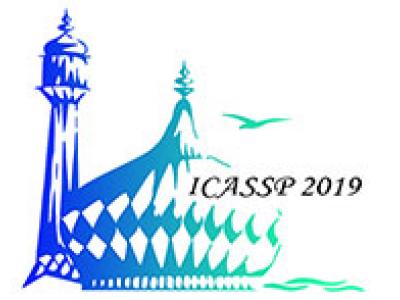
- Read more about 3D Coprime Arrays in Sparse Sensing
- Log in to post comments
icassp_s09.pdf

- Categories:
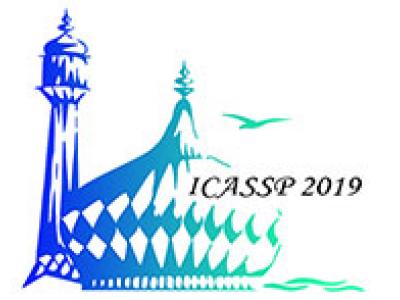
- Read more about Robust Bayesian Beamforming for Sources at Different Distances with Applications in Urban Monitoring
- Log in to post comments
Poster for "Robust Bayesian Beamforming for Sources at Different Distances with Applications in Urban Monitoring".
https://ieeexplore.ieee.org/document/8682835
poster.pdf

- Categories:
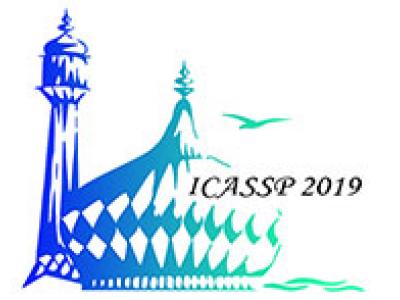
- Read more about AN LS LOCALISATION METHOD FOR MASSIVE MIMO TRANSMISSION SYSTEMS
- Log in to post comments
We present a novel localization method based on directional beams,
as available in novel massive MIMO transmission techniques instead
of radius information, and derive a least squares (LS) estimation
method. The new method is a direct LS method that can be solved by
a linear set of equations rather than an iterative method required for
radius information. In a further step, we also show how to transform
radius information into virtual beams to apply the proposed method.
Finally, we evaluate the accuracy of the new methods by simulations.
poster_v2.pdf

- Categories: