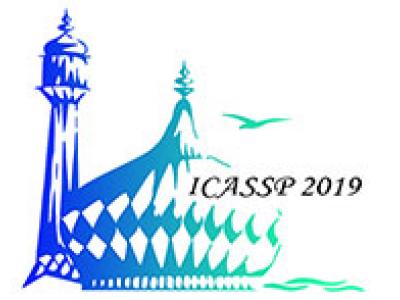
- Read more about QUESTION ANSWERING FOR SPOKEN LECTURE PROCESSING
- Log in to post comments
This paper presents a question answering (QA) system developed for spoken lecture processing. The questions are presented to the system in written form and the answers are returned from lecture videos. In contrast to the widely studied reading comprehension style QA – the machine understands a passage of text and answers the questions related to that passage – our task introduces the challenge of searching the answers on longer text where the text corresponds to the erroneous transcripts of the lecture videos.
- Categories:
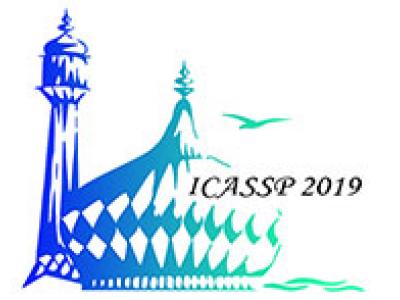
- Read more about QUESTION ANSWERING FOR SPOKEN LECTURE PROCESSING
- Log in to post comments
This paper presents a question answering (QA) system developed for spoken lecture processing. The questions are presented to the system in written form and the answers are returned from lecture videos. In contrast to the widely studied reading comprehension style QA – the machine understands a passage of text and answers the questions related to that passage – our task introduces the challenge of searching the answers on longer text where the text corresponds to the erroneous transcripts of the lecture videos.
- Categories:
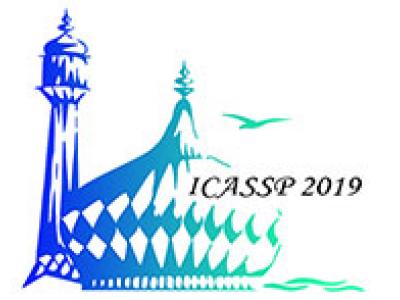
- Read more about REVISITING HIDDEN MARKOV MODELS FOR SPEECH EMOTION RECOGNITION
- Log in to post comments
- Categories:
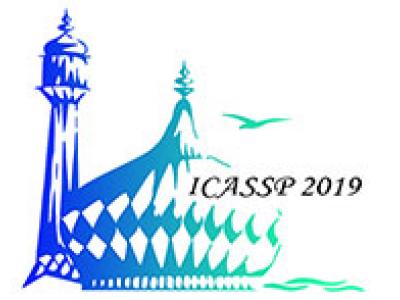
- Read more about USING DEEP-Q NETWORK TO SELECT CANDIDATES FROM N-BEST SPEECH RECOGNITION HYPOTHESES FOR ENHANCING DIALOGUE STATE TRACKING
- Log in to post comments
- Categories:
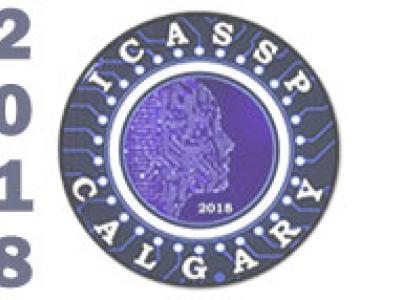
- Read more about Adversarial Advantage Actor-Critic Model for Task-Completion Dialogue Policy Learning
- Log in to post comments
This paper presents a new method --- adversarial advantage actor-critic (Adversarial A2C), which significantly improves the efficiency of dialogue policy learning in task-completion dialogue systems. Inspired by generative adversarial networks (GAN), we train a discriminator to differentiate responses/actions generated by dialogue agents from responses/actions by experts.
- Categories:
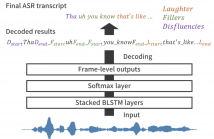
- Read more about AN END-TO-END APPROACH TO JOINT SOCIAL SIGNAL DETECTION AND AUTOMATIC SPEECH RECOGNITION
- Log in to post comments
Social signals such as laughter and fillers are often observed in natural conversation, and they play various roles in human-to-human communication. Detecting these events is useful for transcription systems to generate rich transcription and for dialogue systems to behave as we do such as synchronized laughing or attentive listening. We have studied an end-to-end approach to directly detect social signals from speech by using connectionist temporal classification (CTC), which is one of the end-to-end sequence labelling models.
- Categories:
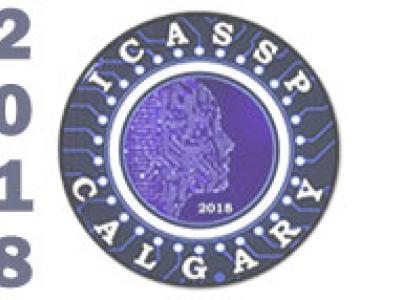
- Read more about Incorporating ASR Errors with Attention-based, Jointly Trained RNN for Intent Detection and Slot Filling
- Log in to post comments
- Categories:
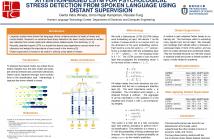
- Read more about ATTENTION-BASED LSTM FOR PSYCHOLOGICAL STRESS DETECTION FROM SPOKEN LANGUAGE USING DISTANT SUPERVISION
- Log in to post comments
- Categories:
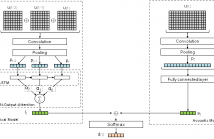
- Read more about Lexico-acoustic Neural-based Models for Dialog Act Classification
- Log in to post comments
Recent works have proposed neural models for dialog act classification in spoken dialogs.
However, they have not explored the role and the usefulness of acoustic information.
We propose a neural model that processes both lexical and acoustic features for classification.
Our results on two benchmark datasets reveal that acoustic features are helpful in improving the overall accuracy.
- Categories:
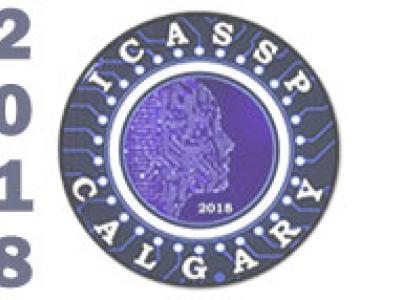
- Read more about Joint Verification-Identification in End-to-End Multi-Scale CNN Framework for Topic Identification
- Log in to post comments
We present an end-to-end multi-scale Convolutional Neural
Network (CNN) framework for topic identification (topic ID).
In this work, we examined multi-scale CNN for classification
using raw text input. Topical word embeddings are learnt at
multiple scales using parallel convolutional layers. A technique
to integrate verification and identification objectives is
examined to improve topic ID performance. With this approach,
we achieved significant improvement in identification
task. We evaluated our framework on two contrasting
- Categories: