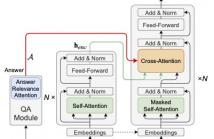
- Read more about QA4QG: USING QUESTION ANSWERING TO CONSTRAIN MULTI-HOP QUESTION GENERATION
- Log in to post comments
Multi-hop question generation (MQG) aims to generate complex questions which require reasoning over multiple pieces of information of the input passage. Most existing work on MQG has focused on exploring graph-based networks to equip the traditional Sequence-to-sequence framework with reasoning ability. However, these models do not take full advantage of the constraint between questions and answers. Furthermore, studies on multi-hop question answering (QA) suggest that Transformers can replace the graph structure for multi-hop reasoning.
- Categories:
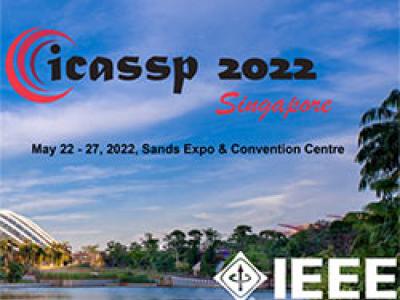
- Read more about Knowledge Augmented BERT Mutual Network in Multi-turn Spoken Dialogues
- Log in to post comments
- Categories:
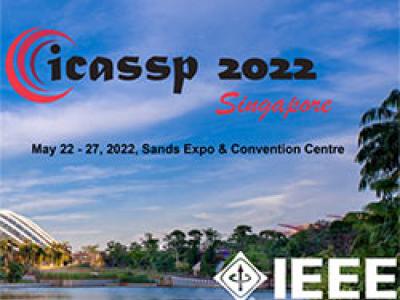
- Read more about ESPNET-SLU: ADVANCING SPOKEN LANGUAGE UNDERSTANDING THROUGH ESPNET
- Log in to post comments
5372-1.pdf

- Categories:
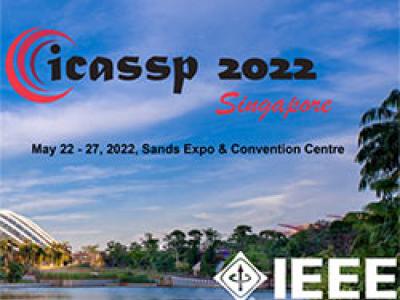
Emotion recognition in conversations (ERC) has attracted increasing interests in recent years, due to its wide range of applications, such as customer service analysis, health-care consultation, etc. One key challenge of ERC is that users' emotions would change due to the impact of others' emotions. That is, the emotions within the conversation can spread among the communication participants. However, the spread impact of emotions in a conversation is rarely addressed in existing researches.
- Categories:
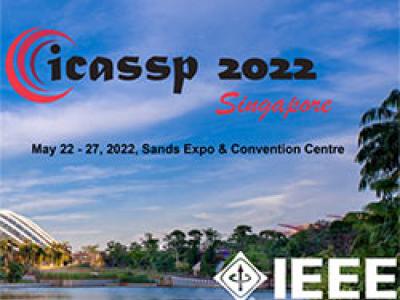
- Read more about Towards End-to-End Integration of Dialog History for Improved Spoken Language Understanding
- Log in to post comments
Dialog history plays an important role in spoken language understanding (SLU) performance in a dialog system. For end-to-end (E2E) SLU, previous work has used dialog history in text form, which makes the model dependent on a cascaded automatic speech recognizer (ASR). This rescinds the benefits of an E2E system which is intended to be compact and robust to ASR errors. In this paper, we propose a hierarchical conversation model that is capable of directly using dialog history in speech form, making it fully E2E.
- Categories:
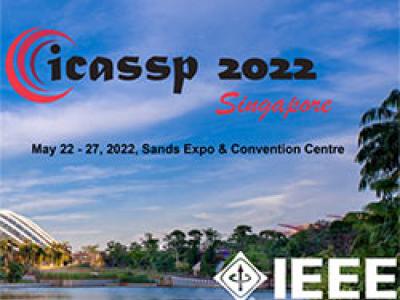
- Read more about NEWS RECOMMENDATION VIA MULTI-INTEREST NEWS SEQUENCE MODELLING
- Log in to post comments
A session-based news recommender system recommends the next news to a user by modeling the potential interests embedded in a sequence of news read/clicked by her/him in a session. Generally, a user's interests are diverse, namely there are multiple interests corresponding to different types of news, e.g., news of distinct topics, within a session. However, most of existing methods typically overlook such important characteristic and thus fail to distinguish and model the potential multiple interests of a user, impeding accurate recommendation of the next piece of news.
- Categories:
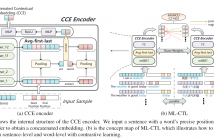
- Read more about MULTI-LEVEL CONTRASTIVE LEARNING FOR CROSS-LINGUAL ALIGNMENT
- Log in to post comments
Cross-language pre-trained models such as multilingual BERT (mBERT) have achieved significant performance in various cross-lingual downstream NLP tasks. This paper proposes a multi-level contrastive learning (ML-CTL) framework to further improve the cross-lingual ability of pre-trained models. The proposed method uses translated parallel data to encourage the model to generate similar semantic embeddings for different languages.
- Categories:
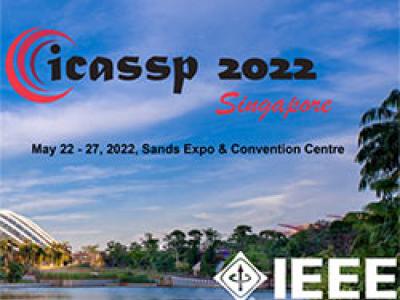
- Read more about Robust Unstructured Knowledge Access In Conversational Dialogue With ASR Errors
- Log in to post comments
Performance of spoken language understanding (SLU) can be degraded with automatic speech recognition (ASR) errors. We propose a novel approach to improve SLU robustness by randomly corrupting clean training text with an ASR error simulator, followed by self-correcting the errors and minimizing the target classification loss in a joint manner. In the proposed error simulator, we leverage confusion networks generated from an ASR decoder without human transcriptions to generate variety of error patterns for model training.
- Categories:
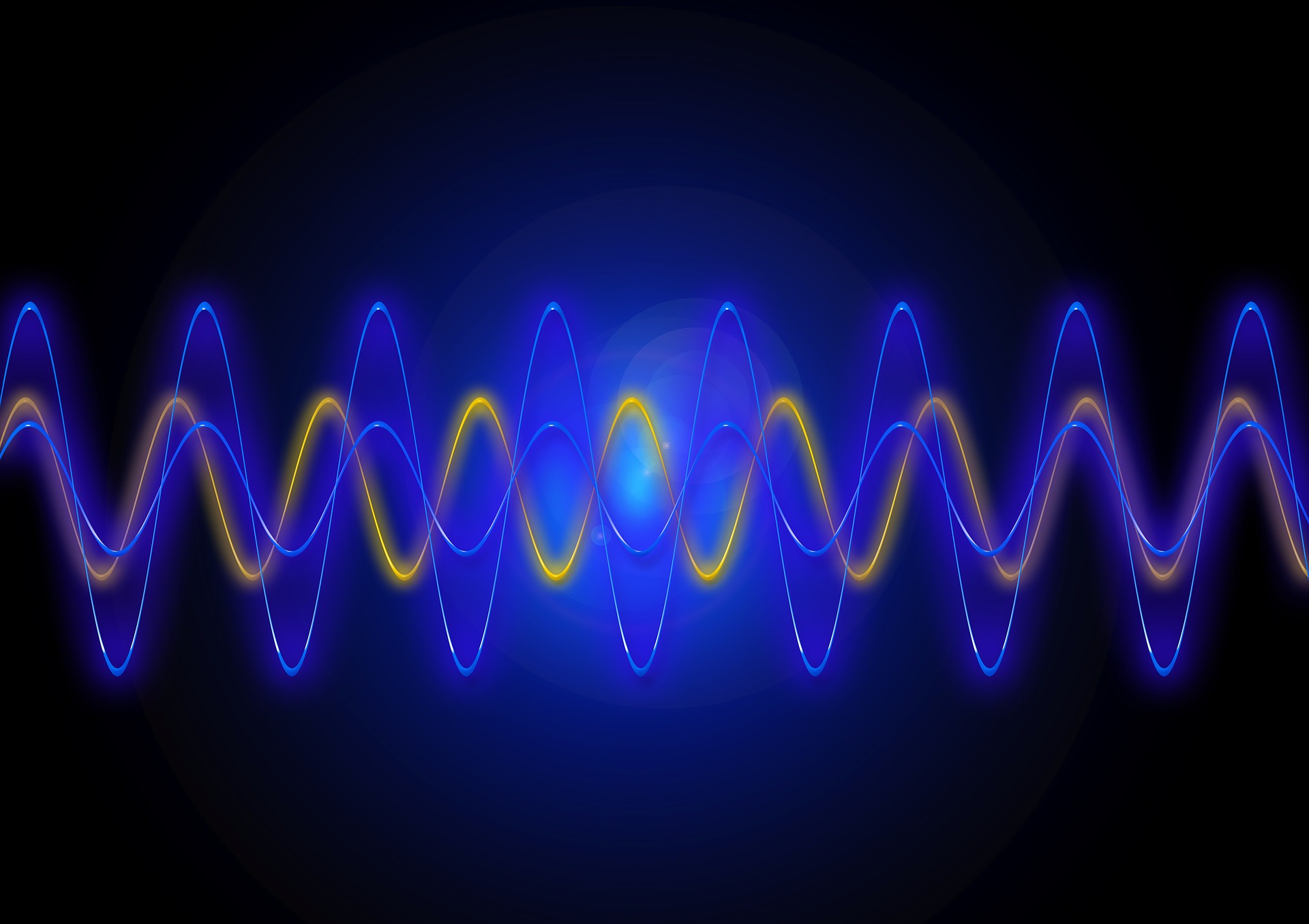
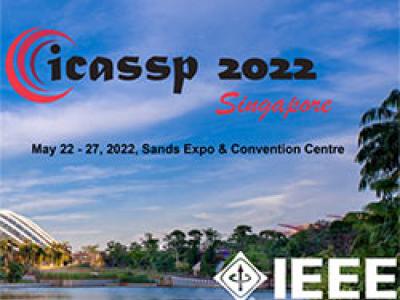