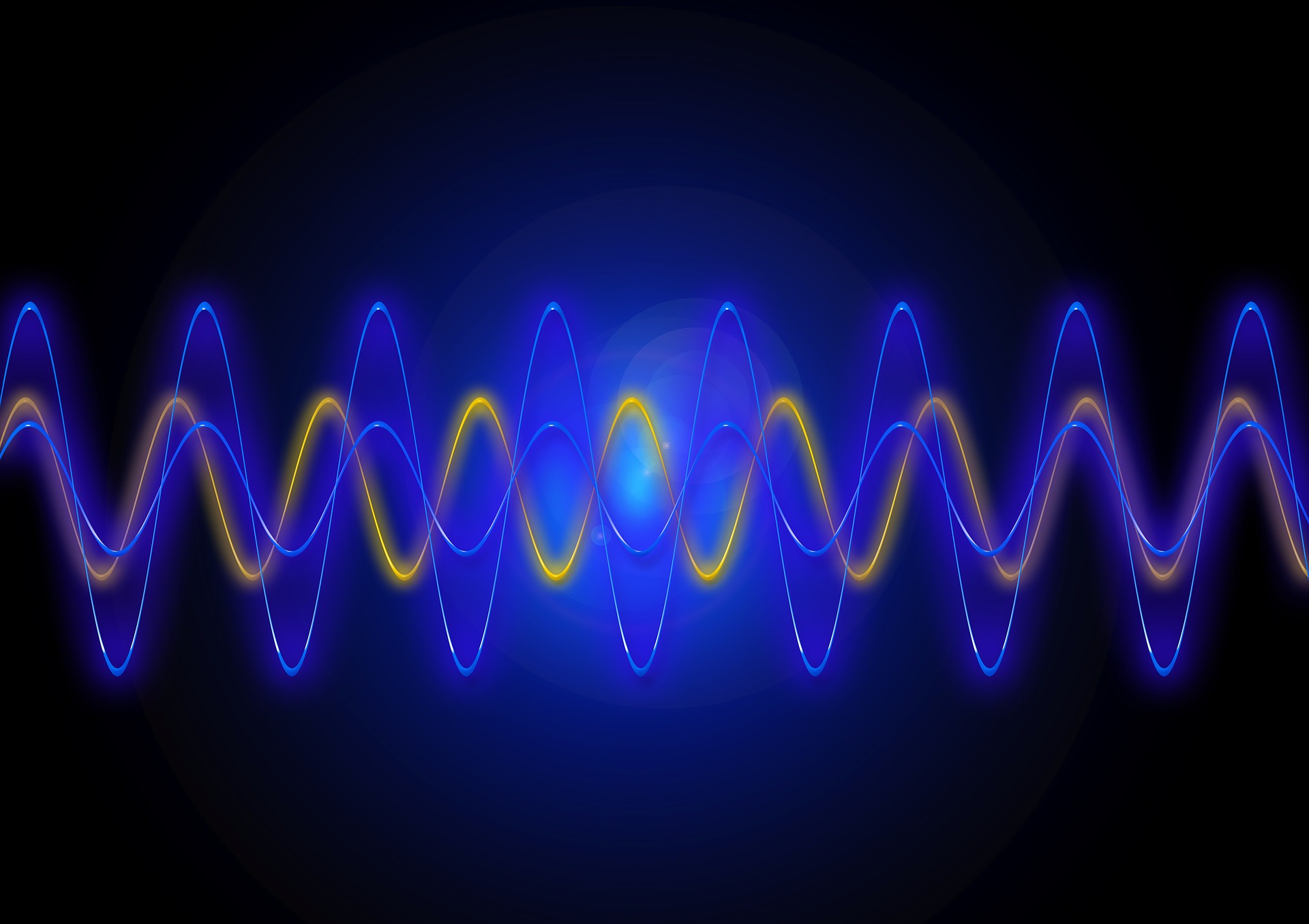
- Read more about Supplementary material S3VD self-supervised spatial downsampling loss: A method for training video FPN denoising networks
- Log in to post comments
Fixed pattern noise (FPN) is a temporally constant noise present on videos due to the non-uniformities of the sensors that may exhibit spatial correlation, typically across columns and/or rows.
Acquiring real clean/noisy data is particularly challenging in the case of FPN, leading supervised FPN denoising networks to train using generated data.
Self-supervised approaches for denoising allow training directly on real noisy sequences, avoiding the biases introduced by synthetic data.
- Categories:
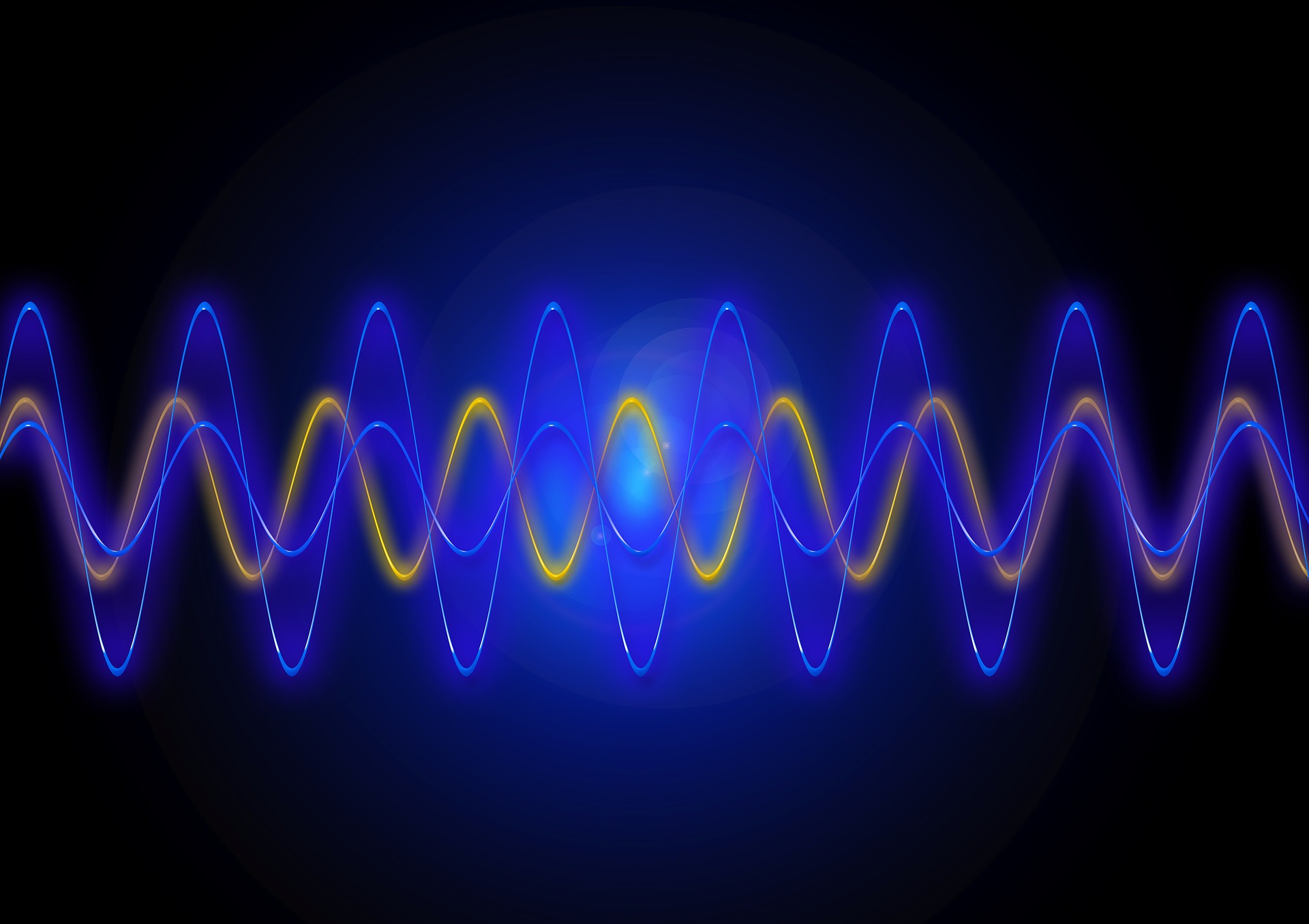
- Read more about Supplementary Materials
- Log in to post comments
Video supplementary materials
- Categories:
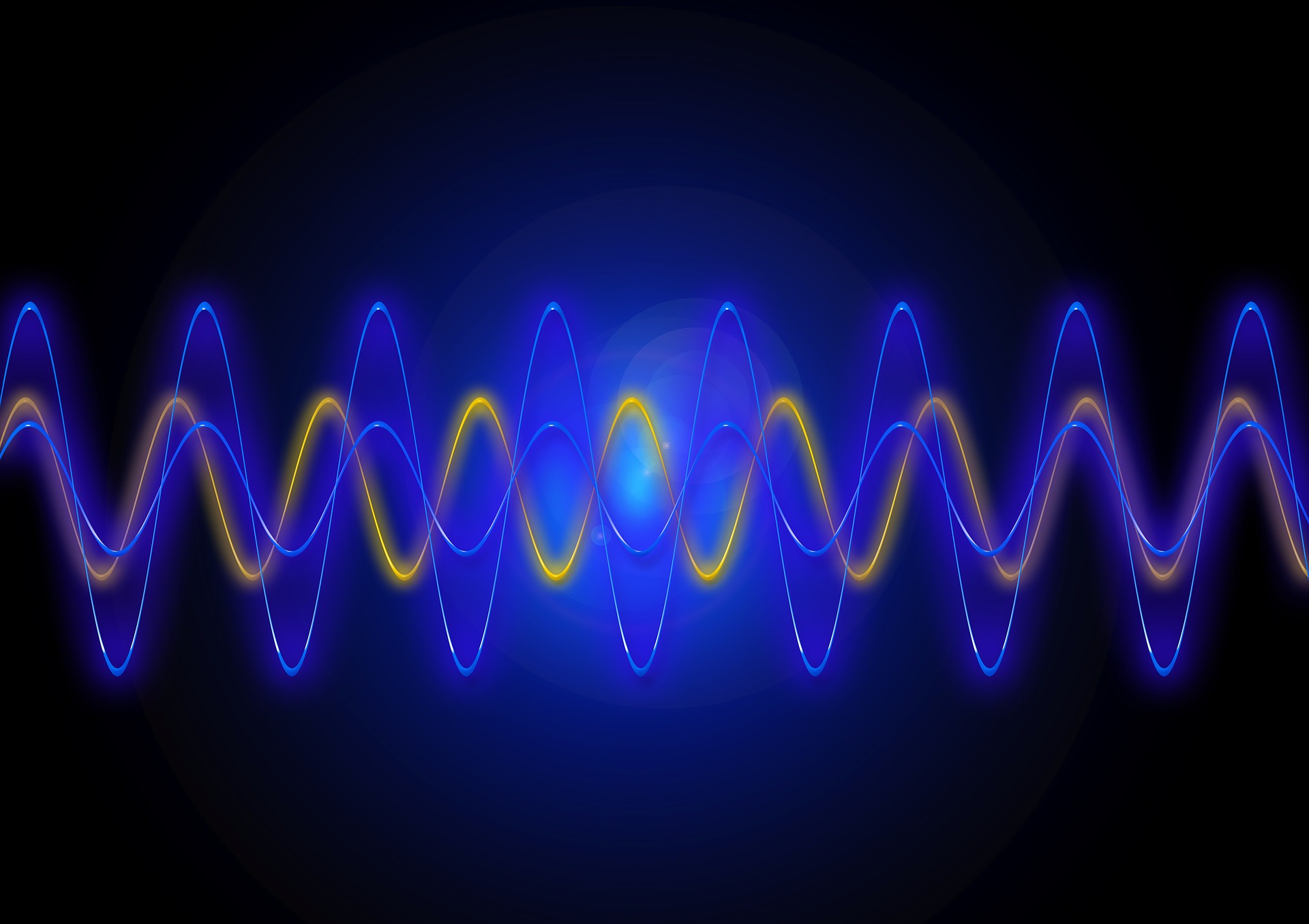
- Read more about Supplementary material - GeoScaler
- Log in to post comments
GEOSCALER: GEOMETRY AND RENDERING-AWARE DOWNSAMPLING OF 3D MESH TEXTURES: High-resolution texture maps are necessary to accurately represent real-world objects with 3D meshes. The large sizes of textures can bottleneck the real-time rendering of high-quality virtual 3D scenes on devices that have low computational budgets and limited memory. Downsampling the texture maps directly addresses the issue, albeit at the cost of visual fidelity. Traditionally, downsampling of texture maps is performed using methods such as bicubic interpolation and the Lanczos algorithm.
- Categories:
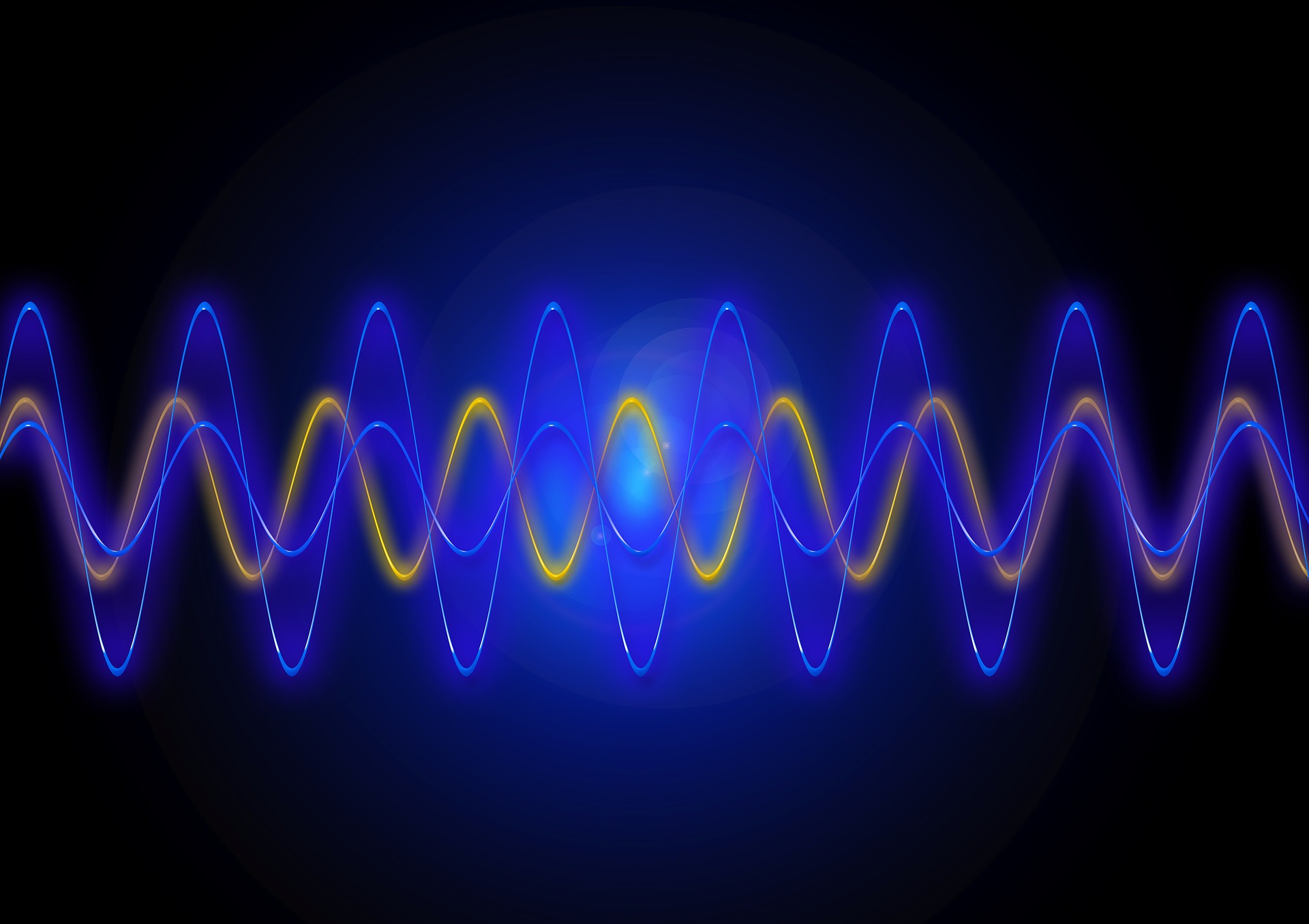
- Read more about SUPPLEMENTARY MATERIAL FOR “A UNIFIED FRAMEWORK FOR DYNAMIC POINT CLOUD COMPRESSION”
- Log in to post comments
Point cloud compression is a critical component in 3D vision systems utilizing point cloud data to represent the physical world. Existing works on point cloud compression separately tackle Octree-based and Feature-based compression of point clouds despite their underlying similarities. In this work, we present UniFHiD: a unified fully hierarchical model for dynamic point cloud compression, that synergizes Octree and Feature Coding under a single model with shared parameters. The key enabler in this unification is the aligned feature extraction between the two coding methods.
- Categories:
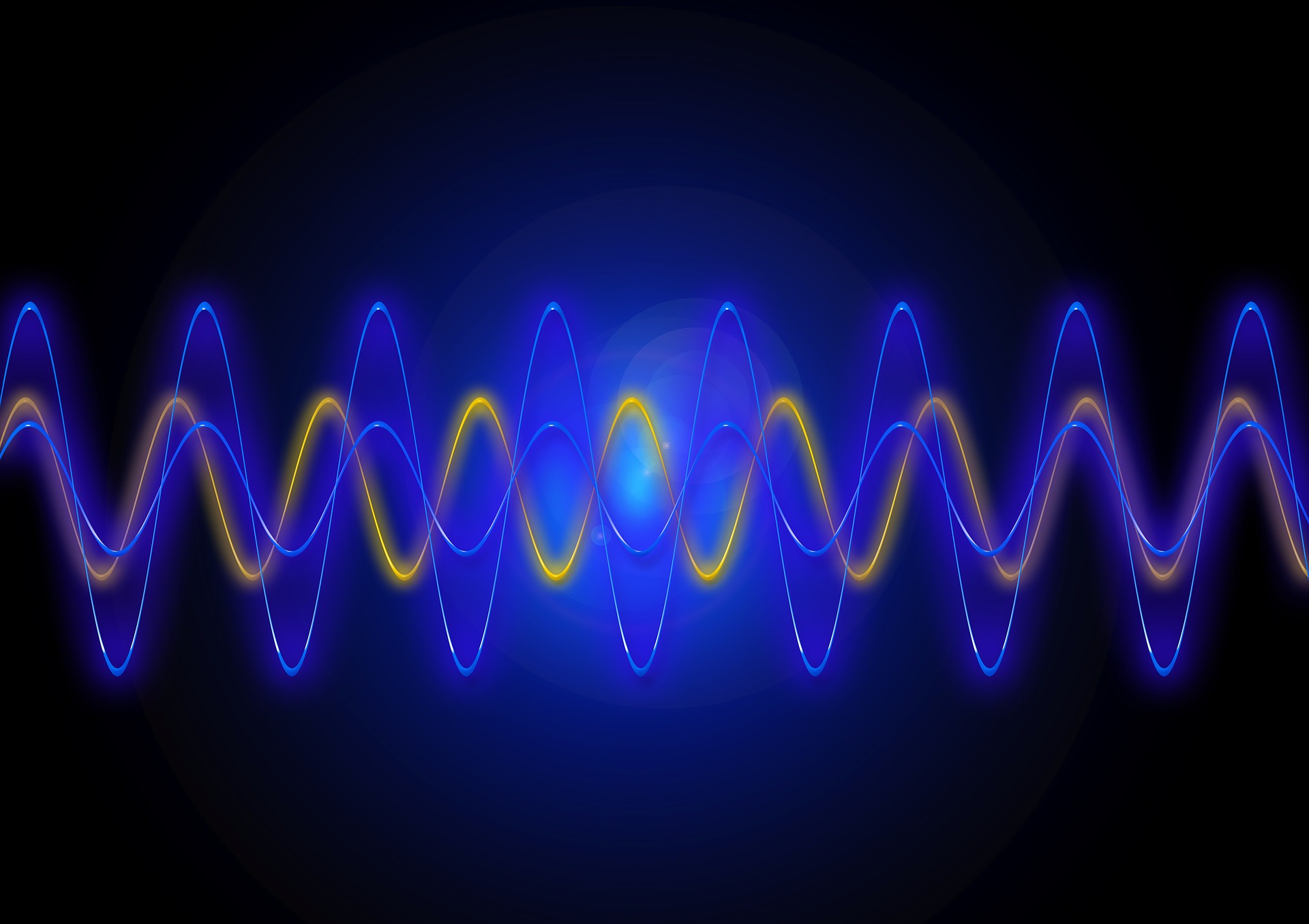
- Read more about SUPPLEMENTARY: LEVERAGING 3D GAUSSIAN SPLATTING TO ENHANCE FACE PARSING
- Log in to post comments
This is supplementary to our paper titled: LEVERAGING 3D GAUSSIAN SPLATTING TO ENHANCE FACE PARSING
- Categories:
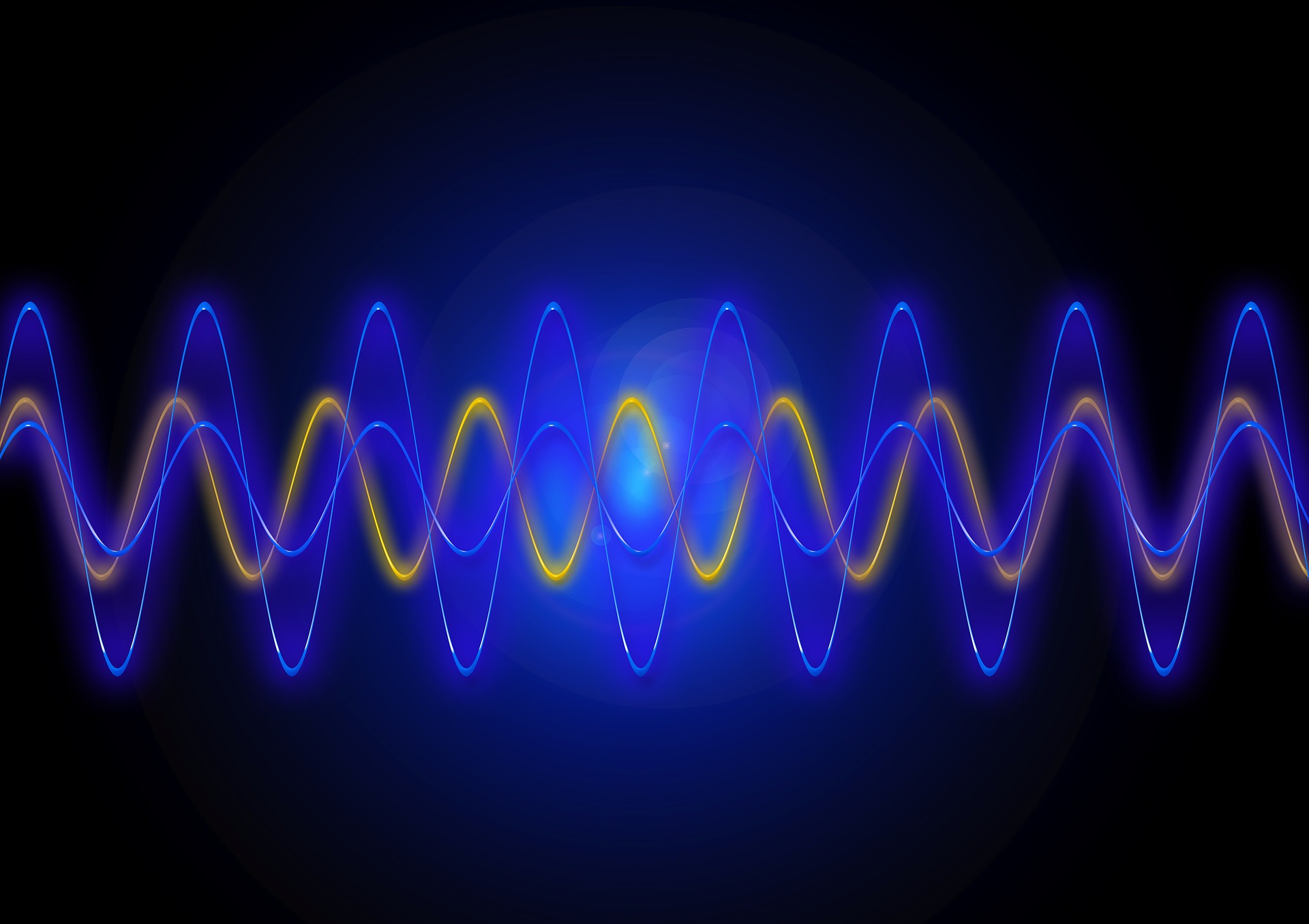
- Read more about MS-RAFT-3D_supplementary
- Log in to post comments
This document is the supplementary material of our submitted paper: MS-RAFT-3D: A Multi-Scale Architecture for Recurrent Image-based Scene Flow.
- Categories:
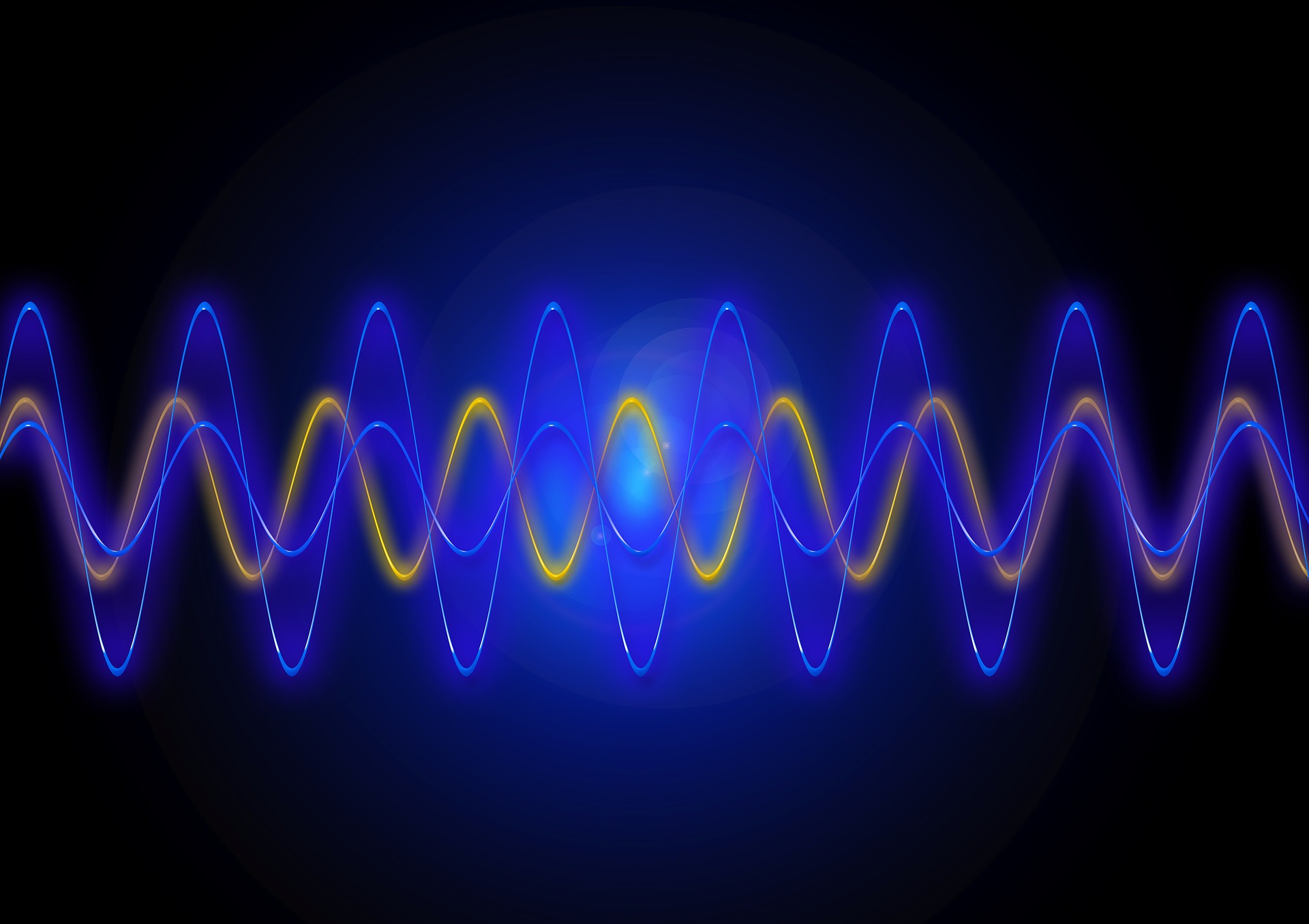
- Read more about SPHERICAL DENSE TEXT-TO-IMAGE SYNTHESIS
- Log in to post comments
Recent advancements in text-to-image (T2I) have improved synthesis results, but challenges remain in layout control and generating omnidirectional panoramic images. Dense T2I (DT2I) and spherical T2I (ST2I) models address these issues, but so far no unified approach exists. Trivial approaches, like prompting a DT2I model to generate panoramas can not generate proper spherical distortions and seamless transitions at the borders. Our work shows that spherical dense text-to-image (SDT2I) can be achieved by integrating training-free DT2I approaches into finetuned panorama models.
- Categories:
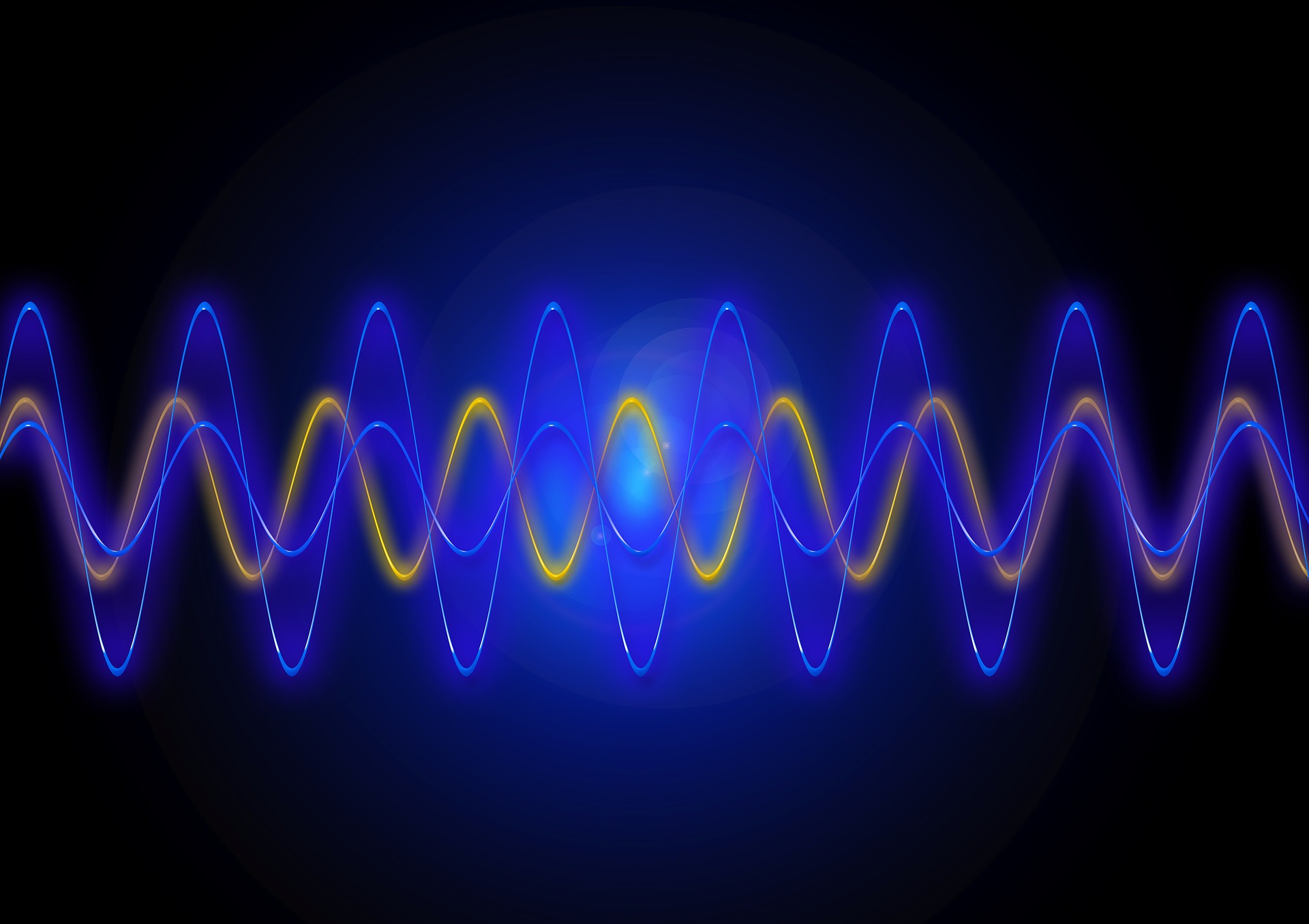
- Read more about Supp of D&R
- Log in to post comments
In this supplementary material, we provide implementation details of our method, training details of our method, additional experiments and visual results.
SuppDR.pdf

- Categories:
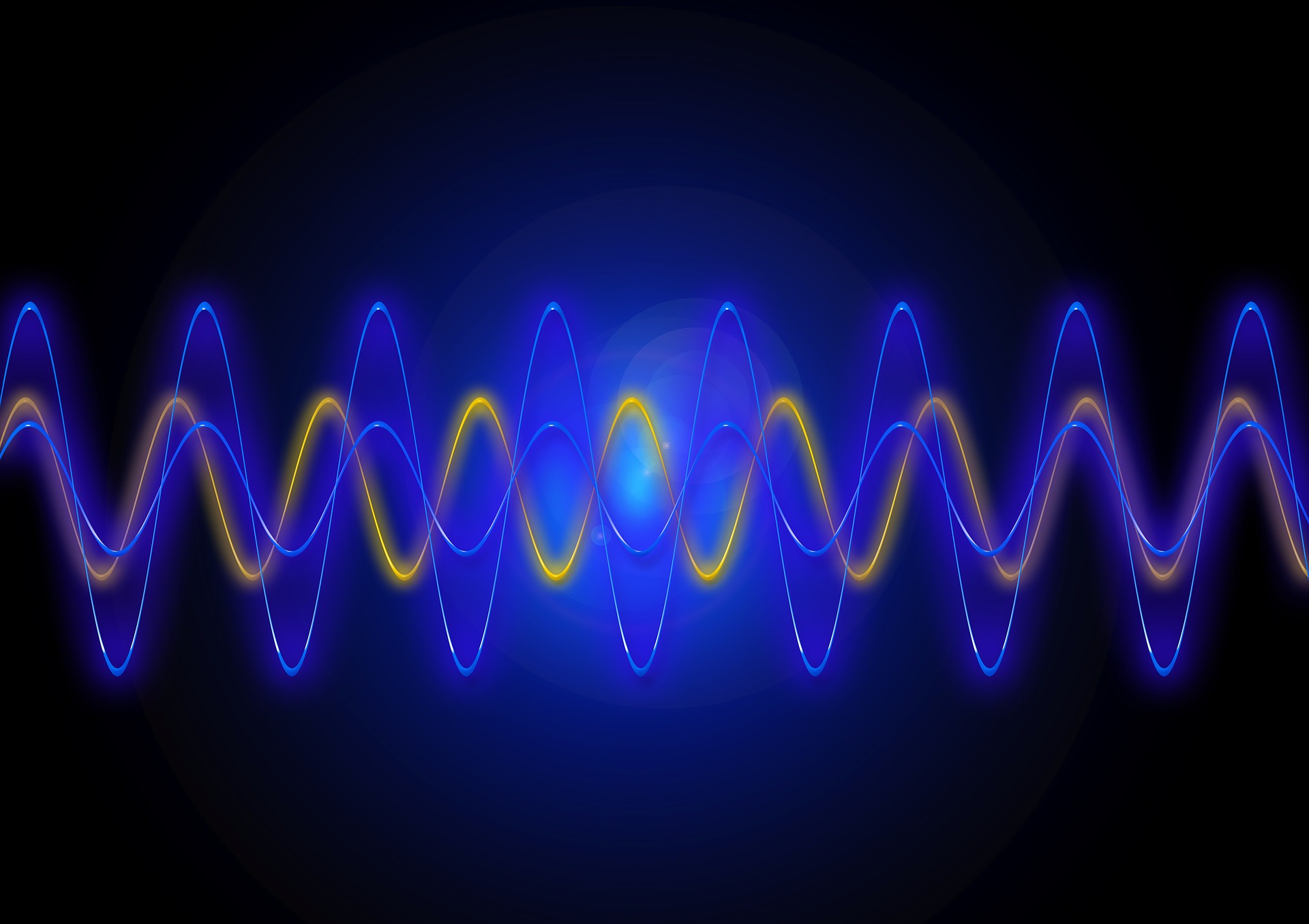
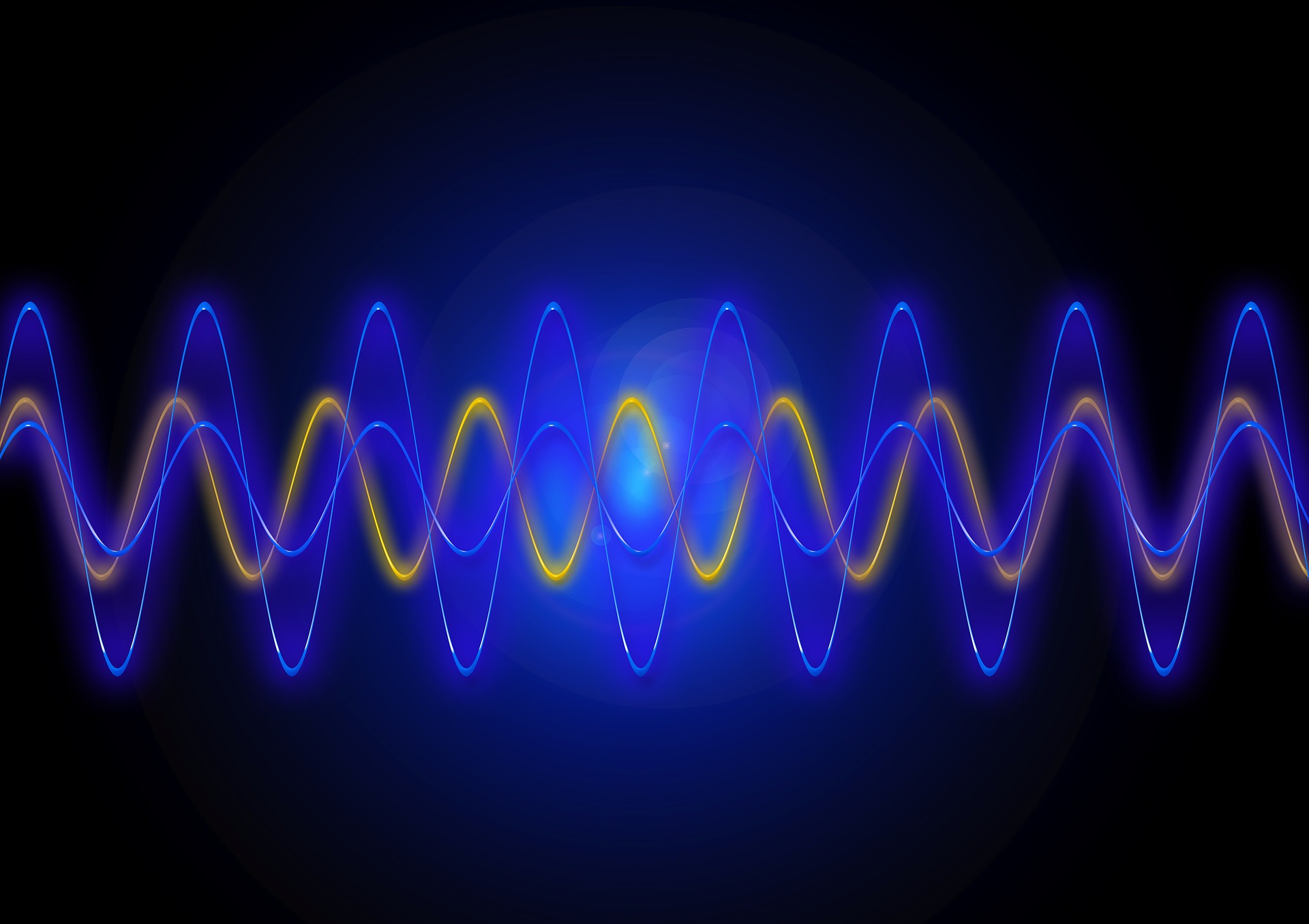
- Read more about Supplementary Materials for the paper "A COLOR PRESERVING TONE MAPPING FRAMEWORK IN THE INTRINSIC DOMAIN"
- Log in to post comments
Tone mapping is an essential step in an acquisition or a rendering pipeline to map high dynamic range (HDR) content to a reference display range. The simplest tone mapping approach is to apply a function to the luminance channel of an HDR image and then to propagate the change to the red, green, and blue channels. However, this often causes color distortions since luminance and chrominance channels are interdependent, and modifying one affects the other.
- Categories: